Development of a Scale to Measure Planned Behavior in Inclusive Science Communication: Validity Evidence in Undergraduate STEM Students
Abstract
Science communication has historically been inequitable, with certain voices and perspectives holding the power and dominant ways of knowing being promoted over others. Recently, there has been a push toward inclusive science communication, which values diverse perspectives and ways of knowing in collaborative conversations to solve complex socioscientific issues. However, there is a lack of both trainings in inclusive science communication for undergraduate science, technology, engineering, and mathematics (STEM) students as well as established ways to evaluate the efficacy of these trainings. To address this need, we designed a new multifactorial survey based on the Theory of Planned Behavior to assess students’ attitudes/norms, self-efficacy, behavioral intents, and behaviors in inclusive science communication, which we termed the Planned Behaviors in Inclusive Science Communication (PB-ISC) Scale. We utilized expert review, exploratory factor analysis, confirmatory factor analysis, cognitive interviews, and quantitative measures to gather evidence of validity supporting the proposed use of the final 4-factor, 26-item survey. This survey can be used as a tool by science communication educators and researchers to assess students’ planned behavior in inclusive science communication in response to trainings or experiences in science communication or related topics like socioscientific issues, civic engagement, and citizen science.
INTRODUCTION
Inclusive Approaches to Science Communication as a Means to Expand Justice in STEM
Traditionally, science communication has focused on deficit approaches, with scientists being considered the rational experts and nonscientists considered as an ignorant monolith with a deficit of knowledge about science (Simis et al., 2016; Suldovsky, 2016). However, more participatory and inclusive approaches to science communication recognize the need for diverse ways of knowing, multidisciplinary perspectives, Indigenous and other non-Western scientific knowledge, and cultural funds of knowledge all being utilized together in order to solve socioscientific issues (Berkes et al., 2000; Trench, 2008; Berkes, 2009; Suldovsky, 2018; Nadkarni et al., 2019; Canfield et al., 2020; Judd and McKinnon, 2021; Callwood et al., 2022; Choi et al., 2023; Vickery et al., 2023). While there is clear evidence on the efficacy of more participatory and inclusive approaches in science communication (O'Mara-Eves et al., 2015; Metcalfe et al., 2022), science communication training and practice for science, technology, engineering, and mathematics (STEM) students and researchers tends to focus on more unidirectional, deficit approaches to science communication (Besley and Tanner, 2011; Besley et al., 2016; Simis et al., 2016; Vickery et al., 2023).
Beyond the need to make science communication training more inclusive for STEM students so that they develop the skillset for inclusive and collaborative science communication in their future careers (Nogueira et al., 2021), inclusive science communication training has an important impact on the students themselves. Training in science communication increases factors such as students’ science identity and science self-efficacy (Cameron et al., 2020; Alderfer et al., 2023), which are correlated with increased STEM persistence, especially for students of historically marginalized backgrounds (Estrada et al., 2011). Because inclusive science communication by definition values the assets provided by people of diverse backgrounds, it is a tool to support students’ consideration of their own and others’ community cultural wealth (Yosso, 2005; Alderfer et al., 2023). Similarly, because inclusive science communication aims to combat the traditionally exclusionary and deficit approaches in Western science (Callwood et al., 2022; Perez et al., 2023), inclusive science communication is a tool to support students in combatting factors like perfectionism and fear of conflict that can hamper their science communication practice and their educational journeys (Alderfer et al., 2023). Overall, an emphasis on inclusivity and justice in our science communication training helps STEM students holding traditionally marginalized identities to consider the assets they themselves provide, while helping students holding traditionally dominant identities to consider the assets of others. Training, practice, and experience in inclusive science communication can help students of excluded identities not just assimilate into the culture of science but actually be empowered to change the culture of science and science communication.
Developing, Implementing, and Evaluating Science Communication Training for STEM Students
Based on the efficacy of inclusive science communication practice as well as the impact of inclusive science communication training on students, it is important to provide more training and experience in inclusive science communication for students. However, most published science communication curricula for STEM undergraduate and graduate students focus on more deficit approaches such as removing jargon rather than more inclusive approaches such as listening to and learning from diverse perspectives (Vickery et al., 2023). Much of the science communication training and practice implemented by STEM faculty does not build upon social science fields (Simis et al., 2016; Suldovsky, 2016) such as psychology, ethnic studies, science communication, and others that inform more just, equitable, and anti-racist practice in science communication. Thus, STEM faculty and students tend to utilize deficit approaches that lack cultural competence and that focus on unidirectional transmission of scientific findings instead of collaboration during the scientific process.
Additionally, there are limited frameworks and scales for evaluating and measuring the efficacy of inclusive approaches to science communication training (Vickery et al., 2023). What is measured is what is valued. If science communication is only measured according to Western science and deficit model approaches, this indicates that only these perspectives are valued. Conversely, if science educators and communicators move toward measuring inclusive mindsets and practices in science communication, more justice-centered approaches toward science and science communication can be appreciated. In this study, we aimed to provide a new tool for science communication educators and researchers to measure more inclusive approaches to science communication. This tool helps move the field of science communication education toward recognition of multiple ways of knowing instead of deficit views toward nonscientists, those with less education, or those from non-Western cultures.
Previous studies have been performed to develop survey constructs for science communication training and students’ perspectives on science communication. For example, the Science Communication Training Effectiveness (SCTE) scale has been validated to measure several constructs related to students’ science communication motivation, self-efficacy, cognition, affect, and behavior (Rodgers et al., 2020). However, the authors recognize that this scale specifically measures the efficacy of a training course to help graduate students explain their research to a nonexpert audience, and “it cannot be concluded that the SCTE scale is the ‘be-all-end-all’ tool” (Rodgers et al., 2020). While it is very important for graduate students to be able to explain their research to various audiences, this is simply one form of science communication and does not emphasize other inclusive forms of science communication such as coproduction between scientists and other interested parties to solve a problem (Nogueira et al., 2021) or boundary spanning conversations by undergraduates of marginalized identities with their families (Couch et al., 2022; Shah et al., 2022). Similarly, the Science Networking Scale focuses on how undergraduate students discuss their course-based undergraduate research with diverse audiences (Hanauer and Hatfull, 2015), but is similarly limited to how a STEM student explains their personal research projects to others, one component but certainly not the entirety of science communication.
The Student Attitudes Towards Communication Skills Survey (SATCSS) measures how undergraduate students value learning communication skills according to Expectancy-Value Theory (Cline et al., 2022). Validity evidence for these survey items was collected with undergraduate students and has a focus on how they perceive the value of verbal, written, and nonverbal communication in their future science careers (Cline et al., 2022). While a focus on use of science communication in a future career can certainly maximize students’ self-assessment of factors, such as confidence in future work selves (Strauss et al., 2012), these scales do not explicitly reference inclusive approaches to science communication.
The Essential Elements for Effective Science Communication (EEES) Framework was developed to incorporate both desired student communication skills according to Vision & Change as well as evidence-based goals for science communication according to the science of science communication literature (Wack et al., 2021). This framework is helpful for evaluating both student plans for science communication as well as their behaviors in science communication via thematic and content analysis (Shivni et al., 2021). While this framework captures important elements in inclusive science communication, it has not been operationalized as a survey scale.
These scales and frameworks provide helpful background for the ways in which science communication training has been previously evaluated, including survey validation techniques and theoretical foundations. In this study, we aimed to build on this foundation by developing a survey scale that more explicitly assessed students’ attitudes toward and behavior in inclusive approaches to science communication. We also aimed to gather validity evidence for the use of these scales (Kane, 1992) to assess student growth in response to inclusive science communication training.
Theory of Planned Behavior in Inclusive Science Communication
We utilized the Theory of Planned Behavior (TPB) to guide development of our multifactor survey. The TPB is a model that integrates how perception of attitudes toward a behavior, social norms about a behavior, and perceived behavioral control impact an individuals’ behavioral intentions as well as behaviors (Ajzen, 1991). The TPB is based on an expectancy-value framework, where an individual's behavior is based on how much they value the task as well as how much they expect to succeed in the task (French and Hankins, 2003). The Expectancy-Value Theory was utilized to guide the SATCSS scale as described previously (Cline et al., 2022) and as a component of a framework for evaluating students’ motivations to engage in reading primary scientific literature, which is just one type of science communication (Chatzikyriakidou and McCartney, 2022). More specifically to TPB, strategic science communication has been conceptualized in terms of planned behavior, wherein scientists’ attitudes, normative beliefs, self-efficacy, and behavioral intentions influence how they engage in public engagement (Besley et al., 2019, 2021; Besley and Dudo, 2022). Related to science communication education research, the TPB has been previously used to conceptualize how graduate students perceptions’ of science communication self-efficacy and behavioral intentions increase after science communication trainings (Copple et al., 2020; Akin et al., 2021) and to assess undergraduate STEM students’ motivations and behaviors in STEM community engagement (Murphy and Kelp, 2023).
With TPB, it is important to consider not only the factors influencing behavioral intentions, but also the behavior that the individual plans to do. We developed items that specifically addressed inclusive mindsets and behaviors toward inclusive approaches to science communication. For example, what behaviors do students plan to do? Do they value listening to and reciprocally learning from people of diverse perspectives (Besley and Downs, 2024)? Reciprocity, intentionality, and reflexivity have been theorized to be key tenets of inclusive science communication (Canfield et al., 2020), so examining whether students plan to engage in these mindsets in order to impact their science communication behaviors is critical. Do they consider socioscientific issues and how inclusive approaches to science could help improve the community (Alam et al., 2023) and redress past harms (Callwood et al., 2022)?
The TPB outlines three main influencers of behavioral intents and behaviors: attitudes toward the behavior, subjective norms, and perceived behavioral control. Attitudes toward a behavior encompasses the beliefs that an individual has about a behavior and their evaluation of the outcome of the behavior (Ajzen, 1991). Attitudes have been shown to be the strongest predictor of some scientists’ willingness to prioritize the behavioral goal of eliciting community members perspectives in their research (Besley and Downs, 2024), which is an important manifestation of inclusive approaches to science communication. Subjective norms refers to an individual's perception of whether others consider the behavior valuable (Ajzen, 1991). For example, an interview study of early-career scientists found that they held differing opinions on whether public engagement is an integral part of a scientists’ professional role, and they did not consider inclusive approaches to science communication in this role (Riley et al., 2022). Thus, in our creation of a multifactorial survey, we conceptualized attitudes, beliefs, and values toward inclusive science communication as well as these social norms toward the behavior in one construct. To assess what students think of inclusive science communication, we considered not only what they personally think, but what they consider the field of science and their peers to value in terms of science communication.
Perceived behavioral control includes the concept of self-efficacy, or the confidence that one can accomplish a behavior (Ajzen, 2002). Self-efficacy as originally conceptualized by Bandura includes components of mastery experience, social modeling, improving emotional states, and verbal persuasion (Bandura, 1997). Self-efficacy was an important component of transitioning Social Learning Theory into Social Cognition Theory (SCT) (Bandura, 1986), and is also now an important component of TPB. The link between SCT and TPB is useful for our study, since behavioral planning for science communication relates to learning of science communication. Additionally, one of the tenets of inclusive science communication is reciprocity (Canfield et al., 2020), and SCT highlights the importance of reciprocal interactions between a person and their environment (Bandura, 1986). Thus, for our survey items measuring perceived behavioral control, we focused on self-efficacy in inclusive and reciprocal/dialogic approaches to science communication.
The factors of attitudes and norms as well as self-efficacy influence the behavioral intentions an individual has. It is important to analyze the intentions behind science communication behaviors, since planned science communication is more strategic and effective (Besley et al., 2019) and intentional science communication is more inclusive (Canfield et al., 2020) Understanding the factors influencing scientists’ intentions in science communication can also reveal important mindset issues (Choi et al., 2023). However, there can be discrepancies between behavioral intentions and actual behaviors (Sheeran and Webb, 2016), with effective interventions causing a medium-to-large effect on intentions and a small-to-medium effect on behavior (Webb and Sheeran, 2006). Fortunately, stronger intentions have been shown to be more stable and better predictors of behavior (Conner and Norman, 2022), so working to more greatly increase STEM students’ and scientists’ behavioral intentions toward engaging in inclusive science communication will have a larger impact on their eventual behavior. Measuring intentions can be useful as a more immediate metric of intervention efficacy, and longitudinal assessment of both intentions and behaviors can be useful to assess maintenance of student growth in response to science communication training.
Overall, in this study we aimed to develop a multifactorial scale to measure key constructs related to planned behavior in inclusive science communication. We gathered validity evidence for use of this scale for undergraduate STEM students using expert review, confirmatory factor analysis (CFA) and exploratory factor analysis (EFA), cognitive interviews with members, and comparison of scale metrics as pre/post measurement after inclusive science communication training. This survey is designed to be a tool to evaluate the efficacy of future training and experience in inclusive science communication, socioscientific issues, civic engagement, citizen science, and other related issues. This survey can assess how such trainings and experiences impact students’ planned behaviors in inclusive science communication as a means by which to collaborate to solve complex socioscientific issues.
METHODS AND RESULTS
We followed a collection of methodological resources regarding construct validity (Messick, 1995), including (American Educational Research Association et al., 2014; Knekta et al., 2019; Reeves and Marbach-Ad, 2016) for guidelines on developing a survey and gathering validity evidence for inferences drawn from the proposed use of the survey scale. We also referred to examples of similar scale development, such as the Predictors of Science Civic Engagement (PSCE) survey (Alam et al., 2023). In brief, we performed initial item generation, expert review, EFA, CFA, cognitive interviews, and pilot implementation of the scale to assess changes in student responses to the scale after a science communication training. Overall, we aimed to gather evidence for the validity of inferences that can be drawn from the quantitative scores provided by students’ responses to this self-report attitudinal and behavioral scale (American Educational Research Association et al., 2014). Such evidence is necessary for this scale to be a useful instrument for instructors and science communication education researchers. This study was approved by the Institutional Review Board of Colorado State University, and students consented to their survey responses being used for research.
Initial Scale Development and Expert Review
Author N.C.K. developed the scale based on literature on the topics of inclusive science communication, TPB, and socioscientific issues. We utilize Sadler's definition of socioscientific issues, which are real-world societal problems informed by science and often including controversial, equity, or ethical considerations (Sadler et al., 2007). Initial scale items are listed in Table 1, along with information about the results from the EFA and CFA as described below.
![]() |
Discussion with undergraduate and graduate student researchers in science communication education served as expert review of the scale, which is a form of validity evidence based on test content (Reeves and Marbach-Ad, 2016). The expert review panel (n = 8 individuals) was comprised of STEM student researchers in the field of science communication education research. These individuals have expertise from both their research experience as well as their lived experiences as STEM students who are the intended survey participant population (Beames et al., 2021; National Academies of Sciences et al., 2023; Vázquez et al., 2023) as well as expertise in the discipline of science communication research. Additionally, these students were both life sciences and engineering majors, helping ensure transferability of the survey across STEM fields. During the expert review process, the research team read through each survey item and discussed their interpretation of the item. More than half of the reviewers found the item “I think that scientists make the best decisions about solving socioscientific issues” to be vague, based on the perspective that “best” can be interpreted in multiple ways. Thus, this item was removed based on this discussion. No other items were considered by multiple expert reviewers to be problematic.
EFA and CFA
To provide evidence for validity based on internal structure, we performed EFA and CFA according to established practices (Watkins, 2018; Knekta et al., 2019, 2020).
A survey was built in the online, secure Qualtrics environment. All items were presented in the order listed in Table 1, and instructions were provided as described in the first column of Table 1. All response points were labeled for the Likert scale.
For the EFA, we had n = 598 responses from undergraduate STEM students from a variety of upper- and lower-level classes across the life sciences and engineering at a large R1 land grant university. The students were recruited to complete the survey from disciplinary STEM courses in which the instructor had invited author N.C.K. to do a guest lecture on science communication, but the courses were not otherwise focused on science communication and the surveys were not administered immediately after the training in order to avoid impact of training on student responses. The surveys were administered in five courses (three life sciences courses, two biomedical engineering courses) across two semesters. Response rates from each course varied from 50% to 90%. Students were compensated via a $10 gift card for completing the survey. The demographics of the EFA data included: 46.9% responses from engineering majors and 53.1% responses from life sciences majors; 19.5% responses from students in upper-division courses and 80.5% responses from students in lower-division courses; and 35.5% responses from marginalized students (students identifying as Black, Indigenous, or Person of Color [BIPOC], low socioeconomic status, and/or first-generation college students) and 64.5% responses from students not identifying in one or more of these categories.
Barlett's test for sphericity (Bartlett, 1951) indicated a nonrandom correlation matrix (χ2(378) = 15425.6, p = < 0.0001). The Kaiser–Meyer–Olkin (KMO) statistic (Kaiser, 1974) was 0.90, indicating that the data were an excellent candidate for factor analysis. Analyses were conducted with the open source software R version 4.3.1 (2023-06-16 ucrt) (R Core Team, 2023). Finding number of factors to use and construction of the models were conducted using the nFactors package version 2.4.1.1 and the psych package version 2.3.6. The correlation matrix was created using the polycor package version 0.8.1. Due to the ordinal nature of the data, responses were coded as factors and a polychoric correlation matrix using pairwise complete observations was used. Analyses were conducted using common factor analysis models, with an iterated principal axis method. Initial communalities were estimated by squared multiple correlations. Because the factors are assumed to be correlated, a Promax (oblique) rotation method was used, with Kaiser normalization. In the exploratory analysis, parallel analysis on the correlation matrix suggested five factors; empirical Bayesian information criterion (BIC), minimum average partial (MAP), and the Kaiser criterion suggested five factors; and theory suggested four factors. For thoroughness, 3-, 4-, and 5-factor solutions were evaluated and a 5-factor solution was chosen based on the parallel analysis and Kaiser criterion (i.e., number of eigenvalues ≥ 1) as well as higher loading factors, lowest BIC and root mean square residual (RMSR), and highest Tucker–Lewis index (TLI). The five factors together explained 68% of the variance. ISC Behavioral Intent accounted for the largest explanation of variance, almost twice that of any other single factor. The EFA allowed us to identify a problem with items Q02 and Q06: these two items did not load onto any of the other factors; had poor internal consistency reliability (Guttman's λ = 0.6); were not theoretically meaningful; and resulted in a factor with fewer than three variables. These criteria led us to remove this factor from the final 4-factor solution. Table 2 shows the pattern coefficient matrix for the five factors after promax rotation, and the communality for each item (the proportion of variance in each item that is explained by the five factors). The larger the communality, the better the model performs for that item. The correlation between PA1 (intent) and PA5 (behavior) is high, as is the correlation between PA2 (beliefs/attitudes/norms) and PA3 (self-efficacy). This is expected based on the TPB. However, correlations were all < 0.70, therefore not so high as to question factors (Table 3).
Item | PA1 | PA2 | PA5 | PA3 | PA4 | Communality |
---|---|---|---|---|---|---|
ISC beliefs/attitudes/norms | ||||||
Q01 | 0.1 | 0.63 | −0.15 | 0.06 | −0.17 | 0.49 |
Q02 | 0.02 | −0.09 | −0.07 | 0.1 | 0.81 | 0.63 |
Q03 | −0.19 | 0.87 | 0.13 | −0.02 | 0.06 | 0.68 |
Q04 | −0.04 | 0.97 | −0.01 | −0.09 | −0.01 | 0.83 |
Q05 | −0.15 | 0.67 | 0.25 | −0.07 | 0.07 | 0.41 |
Q06 | 0.01 | 0 | −0.03 | −0.08 | 0.77 | 0.64 |
Q07 | 0.12 | 0.78 | −0.18 | 0.09 | 0.02 | 0.77 |
Q08 | 0.03 | 0.84 | −0.1 | 0.02 | −0.1 | 0.73 |
Q09 | 0.21 | 0.51 | 0 | 0.17 | 0 | 0.54 |
ISC self-efficacy | ||||||
Q10 | 0.03 | 0.17 | −0.08 | 0.66 | 0.1 | 0.57 |
Q11 | −0.16 | −0.17 | 0.34 | 0.7 | −0.07 | 0.59 |
Q12 | 0.07 | 0.11 | −0.09 | 0.81 | 0.05 | 0.76 |
Q13 | −0.09 | 0.01 | 0.11 | 0.82 | −0.02 | 0.7 |
Q14 | 0.05 | 0.1 | −0.04 | 0.72 | −0.02 | 0.63 |
ISC behavior | ||||||
Q15 | 0.16 | −0.12 | 0.6 | 0.06 | −0.1 | 0.57 |
Q16 | 0.3 | 0 | 0.57 | −0.02 | −0.05 | 0.63 |
Q17 | 0.21 | 0.1 | 0.68 | −0.05 | −0.03 | 0.69 |
Q18 | 0.13 | −0.07 | 0.78 | 0.05 | −0.05 | 0.79 |
Q19 | 0.36 | 0.1 | 0.49 | 0.01 | 0.07 | 0.63 |
Q20 | 0.32 | 0.04 | 0.51 | 0.07 | 0.03 | 0.63 |
Q21 | 0.29 | 0.09 | 0.63 | 0 | 0.11 | 0.73 |
ISC intent | ||||||
Q22 | 0.81 | −0.07 | 0.08 | −0.03 | −0.06 | 0.7 |
Q23 | 0.89 | −0.05 | 0.05 | −0.05 | −0.02 | 0.78 |
Q24 | 0.92 | 0 | 0.03 | −0.04 | 0.01 | 0.84 |
Q25 | 0.83 | −0.17 | 0.09 | 0.05 | −0.03 | 0.75 |
Q26 | 0.9 | 0.07 | −0.02 | −0.05 | 0.02 | 0.79 |
Q27 | 0.85 | 0 | 0.02 | 0.04 | 0.06 | 0.78 |
Q28 | 0.84 | 0.09 | 0.06 | −0.04 | 0.05 | 0.8 |
PA1 | PA2 | PA5 | PA3 | |
---|---|---|---|---|
PA1 | ||||
PA2 | 0.36 | |||
PA5 | 0.61 | 0.08 | ||
PA3 | 0.48 | 0.55 | 0.39 | |
PA4 | −0.09 | 0.13 | −0.16 | −0.19 |
For reliability estimates, while Cronbach's α is popular, it underestimates the reliability of a test and overestimates the first factor saturation. Guttman's λ6 considers the amount of variance in each item that can be accounted for the linear regression of all of the other items (the squared multiple correlation) (Guttman, 1945). As shown in Table 4, reliability is high for all factors except PA4, the two-item factor that was removed before CFA.
α | λ6 | |
---|---|---|
PA1 | 0.96 | 0.96 |
PA2 | 0.91 | 0.91 |
PA3 | 0.88 | 0.87 |
PA4 | 0.78 | 0.64 |
PA5 | 0.93 | 0.93 |
Our average loading for EFA was 0.71. Wolf indicates that CFA for loadings of 0.65 with three factors and six indices per factor requires a sample size of at least 150 for CFA (Wolf et al., 2013). For the CFA, since we had four factors with five to seven indices each, we aimed to exceed this standard. We built another online Qualtrics survey with items in the same order and excluded the two items that were removed after the EFA. We collected n = 378 responses for the CFA from a similar population of undergraduate students across diverse courses and majors. These participants were recruited via the same methods as for the EFA data. Students were recruited from four courses (three life sciences, one biomedical engineering). Response rates for each course ranged from 50% to 75%. Students were compensated with a $10 gift card for completing the survey. The demographics of the CFA data included: 36.2% responses from engineering majors and 63.8% responses from life sciences majors; 15.8% responses from students in upper-division courses and 84.2% responses from students in lower-division courses; and 39.2% responses from marginalized students (students identifying as BIPOC, low socioeconomic status, and/or first-generation college students) and 60.8% responses from students not identifying in one or more of these categories.
For our CFA, the survey items were assigned a priori to presume latent factors. Two CFA models were fit to the data using these four factors, using the lavaan package version 0.6.17 allowing for correlated factors and using a variance standardization method. Missing data were removed by listwise deletion. A model using robust maximum likelihood (RML) estimation, with responses treated as continuous variables, was compared with a model using diagonally weighted least squares (DWLS) estimates, with responses treated as ordinal variables. The RML model was a moderate fit; however, the responses for two of the factors were skewed (beliefs/attitudes/norms was skewed high, as was self-efficacy) and the data failed a test of multivariate normality. The DWLS model performed well as indicated by goodness-of-fit measures: comparative fit index (CFI) = 0.99 (ideal > 0.95), TLI = 0.99 (ideal > 0.95), root mean square error of approximation (RMSEA) = 0.06 (acceptable < 0.08), and standardized root mean square residual (SRMR) = 0.06 (ideal < 0.08). A path diagram with loadings and covariances for the preferred ordinal model (DWLS) is shown in Figure 1, and loadings with standard errors are given in Table 5. The final 4-factor, 26-item scale we termed the Planned Behaviors in Inclusive Science Communication (PB-ISC) Scale.
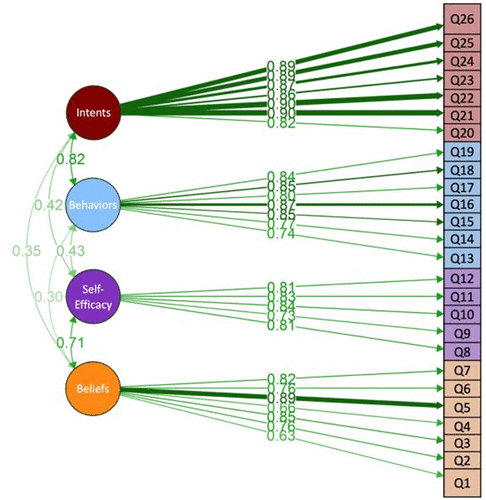
FIGURE 1. CFA path diagram. Path diagram of the ordinal model showing loadings for each item and covariances between factors. Lines are weighted based on size of loading or covariance.
Item | Loading | SE | CI |
---|---|---|---|
ISC Beliefs/attitudes/norms | |||
Q01 | 0.63 | 0.02 | (0.58–0.68) |
Q02 | 0.76 | 0.02 | (0.72–0.80) |
Q03 | 0.85 | 0.02 | (0.80–0.89) |
Q04 | 0.66 | 0.02 | (0.62–0.70) |
Q05 | 0.89 | 0.02 | (0.85–0.93) |
Q06 | 0.76 | 0.02 | (0.72–0.80) |
Q07 | 0.82 | 0.02 | (0.78–0.86) |
ISC Self-efficacy | |||
Q08 | 0.81 | 0.02 | (0.78–0.84) |
Q09 | 0.73 | 0.02 | (0.69–0.76) |
Q10 | 0.84 | 0.02 | (0.81–0.87) |
Q11 | 0.83 | 0.02 | (0.80–0.86) |
Q12 | 0.81 | 0.02 | (0.78–0.84) |
ISC Behaviors | |||
Q13 | 0.74 | 0.01 | (0.72–0.77) |
Q14 | 0.77 | 0.01 | (0.75–0.80) |
Q15 | 0.85 | 0.01 | (0.83–0.87) |
Q16 | 0.87 | 0.01 | (0.85–0.89) |
Q17 | 0.8 | 0.01 | (0.78–0.83) |
Q18 | 0.85 | 0.01 | (0.83–0.87) |
Q19 | 0.84 | 0.01 | (0.82–0.86) |
ISC Behavioral Intents | |||
Q20 | 0.82 | 0.01 | (0.80–0.84) |
Q21 | 0.9 | 0.01 | (0.88–0.92) |
Q22 | 0.9 | 0.01 | (0.89–0.92) |
Q23 | 0.86 | 0.01 | (0.84–0.87) |
Q24 | 0.87 | 0.01 | (0.85–0.89) |
Q25 | 0.89 | 0.01 | (0.87–0.91) |
Q26 | 0.89 | 0.01 | (0.88–0.91) |
Other Validity Evidence: Quantitative
The proposed use of the PB-ISC instrument is to assess changes in the measured factors after science communication, civic engagement, and/or socioscientific issues training. To gather evidence of validity based on consequences of testing, which is evidence for the soundness of proposed interpretation of the scale for its intended use (American Educational Research Association et al., 2014; Reeves and Marbach-Ad, 2016), we utilized a sample of n = 112 students (pooled across two semesters) who had participated in an introduction to inclusive science communication workshop as described by (Alderfer et al., 2023). Briefly, this workshop included discussion about models of science communication from deficit to inclusive, analysis of science communication case studies, practicing interdisciplinary communication using a role-play activity, and making a plan to be an inclusive science communicator in the next month. This workshop has been shown to increase student science identity and science self-efficacy survey metrics (Alderfer et al., 2023). To gather validity evidence for the PB-ISC, we analyzed students’ responses to the scale before and after the workshop using paired t tests to assess the pretest and posttest changes in PB-ISC factors. There was a significant increase in each of the factors in the PB-ISC in response to the training (Figure 2). This finding suggests that the PB-ISC is sensitive enough to detect incremental differences in students’ planned behavior in response to training in inclusive science communication, which provides both validity evidence for the proposed use of scale as well as a demonstration of the proposed implementation for biology education researchers and practitioners.
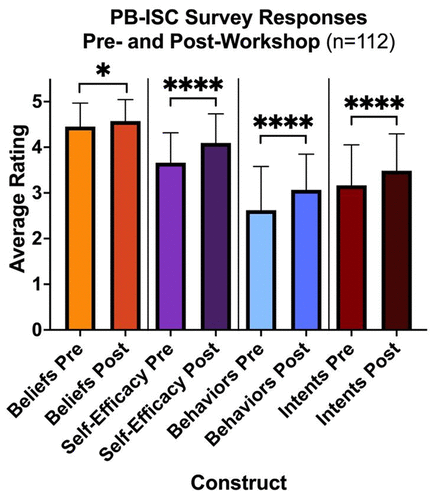
FIGURE 2. Differences in PB-ISC factors before and after a training workshop in inclusive science communication. Results of paired t tests. * = p<0.05, **** = p<0.0001
Because identities are integral to inclusive science communication (Rodrigues et al., 2023), we wanted to assess how students who belong to historically marginalized groups may interpret this scale compared with students belong to historically dominant groups. For the CFA data, we split students who identified as BIPOC, first generation college student, and/or low socioeconomic status (which we collectively termed “marginalized students”) and compared them with students who did not identify in one of these categories (“nonmarginalized students”) using tests for measurement invariance (Cieciuch et al., 2019; Rocabado et al., 2020; Svetina et al., 2020). To establish configural (structural) invariance, the CFA model was fit including a group structure of marginalized (i.e., identifying as BIPOC, first-generation college student, or low socioeconomic status, n = 148) and nonmarginalized respondents (not identifying in any of those categories, n = 230). However, six of the items were empty in one of the two lowest responses in the marginalized group, so responses of “1” and “2” had to be merged into a single level in order to build the model. To assure that our model fit the combined data well, a new CFA model without a group structure was built using the merged responses, and then evaluated for fit statistics (CFA = 0.99, TLI = 0.99, RMSEA = 0.05, SRMR = 0.07). Subsequently, a model built with the group structure was evaluated; the good fit statistics (CFI = 0.99, TLI = 0.99, RMSEA = 0.02, SRMR = 0.06) indicated that the model fit the data across both groups, and that structural invariance was retained (Kline, 2016; Putnick and Bornstein, 2016). For extra confirmation, separate models were fit to each of the groups, and compared. Comparisons of these separate models showed ΔRMSEA = 0.004 and ΔSRMR = 0.02. Additional constrained models were built using the lavaan package and model fits were compared using likelihood ratio tests following recommendations by Vandenberg (Vandenberg and Lance, 2000). Comparisons of a model constrained for group and factor loadings (metric invariance) to that of one constrained for group only failed a likelihood ratio test (Δχ2 = 158.64, df = 22, p < 0.0001; RMSEA = 0.18). Because the p-value is significant, we concluded that weak/metric invariance (equal factor loadings) is not supported in this dataset. Due to lack of metric invariance, scalar invariance testing was not pursued.
Attempts were made to analyze invariance using individual identities (BIPOC [n = 48 BIPOC, n = 327 non-BIPOC], first generation [n = 62 first generation, n = 316 non-first generation], or low socioeconomic status [n = 114 low SES, n = 264 not low SES]) instead of a single “marginalized” group, but the number of empty responses within groups meant that a wide range of answers would have to be combined. We felt that the loss of information in merging answers would result in misleading results and did not proceed further.
Other Validity Evidence: Qualitative
Resources such as (Reeves and Marbach-Ad, 2016; Knekta et al., 2019) indicate that evidence based on response processes includes respondents’ understanding of the scale items as intended by the researcher. To assess this, we performed a combination of solo and dyadic cognitive/think-aloud interviews (Ryan et al., 2012; Morgan et al., 2013; Willis and Artino, 2013). Students (n = 209) were recruited via email from STEM courses in life sciences and engineering who had previously taken the survey for EFA or CFA and consented to be recruited for interviews. In response, n = 5 students were interested in participating in an interview and followed through on scheduling an interview. Researchers D.W. and H.G. performed two dyadic interviews and one solo interview, totaling n = 5 STEM students, who each received a $15 digital gift card after completing the interview process. During the interviews, the researchers showed students the survey items again, asking them which items had resonated with them, why they answered the way they did, whether they found any items confusing, and what they thought these concepts looked like in practice.
Interviews were transcribed, then, D.W. and H.G. utilized emergent coding to identify key themes about the items and use of the items as discussed by students. Across the interviews, feedback about flow, terminology, and purpose of the survey, which yielded no major issues with the survey items but did provide insight for instructors and researchers on implementation of the survey. Students recommended rearranging some of the survey items. For example, in the Behavior section, students recommended arranging the items in the order which they would likely be done (i.e., students are more likely to “think” and “learn” about socioscientific issues before they “discuss” or “explain” them).
Students expressed that the term “socioscientific issue” felt abstract and difficult to define. However, when prompted, students were able to define the term in ways that align with currently established definitions (Sadler et al., 2007). Some examples from students included, “how scientific issues were related to society;” “the humanity of [how science is] gonna affect people;” and “inequality with… different aspects of science… [it is] a social issue affecting people involved with science.” Despite initial hesitation with the term “socioscientific issue,” students did not express issues with their overarching interpretation of the items containing it.
Some students mentioned that while taking the survey, they had difficulty understanding the rationale behind the behavioral intents section which asked them to rank their intended behaviors over the next month. When asked about the frequency of these future conversations, students said “I would say it's more organic and just when something comes up then I'll have a discussion.” Another student responded, “But I don't plan on doing those things… they will just happen if they happen.” These statements were echoed throughout the other interviews and showed how conversations surrounding socioscientific issues may not be regularly planned components of students’ daily lives. This reveals that students potentially have a weakness in planning science communication activities, which presents an opportunity for training students, as we discuss below. However, students did say that they agreed that discussing socioscientific issues is important to do and to think about.
Overall, the cognitive interviews did not reveal any issues with particular items in the PB-ISC, supporting their validity.
DISCUSSION
Summary of Results
In this study, we successfully developed and gathered validity evidence for a multifactorial scale, the PB-ISC, to measure students’ planned behavior and impacts on their behavior regarding inclusive science communication. This PB-ISC scale is useful to assess how students consider the importance of multiple ways of knowing in science and society, which are critical components of increasing justice and changing the culture of STEM. EFA and CFA confirmed four factors based on the TPB constructs of attitudes/norms, self-efficacy, behavioral intents, and behaviors. The factors in the PB-ISC have high factor loadings, and the factors covary as theoretically supported by the TPB. Validity evidence shows theory-supported correlations between these factors, with attitudes/norms and especially self-efficacy correlating with students’ planned behaviors. It is unsurprising that self-efficacy are associated with behaviors more so than attitudes/norms did (i.e., higher covariance, see Figure 1), since perceived behavioral control is theorized to moderate the effects of attitude and norms on behaviors (La Barbera and Ajzen, 2020). Students tended to have higher behavioral intents in inclusive science communication than behaviors, but this is expected based on other studies (Murphy and Kelp, 2023), since students often have desire to participate in science communication but less opportunities to actually do so. Additionally, in focus groups, students revealed that they did not always consciously plan or intend some of their science communication behaviors, but the literature on behavioral intents has highlighted that nonconscious processes are important in behavior change (Papies, 2017). Validity evidence also indicates that these four factors increase in response to training in inclusive science communication, as anticipated. This work contributes to the field of science communication research by continuing the growing emphasis on strategic science communication as planned behavior (Besley et al., 2019; Besley and Dudo, 2022; Besley and Downs, 2024) and contributes to the field of science communication education by creating a survey scale for measuring students’ plans for inclusive science communication, which can be utilized to evaluate the efficacy of science communication trainings (Vickery et al., 2023).
Our cognitive interviews revealed an important need for science communication training, specifically in planning behaviors. While some students said that science communication conversations happen organically, the literature on science communication indicates that planning communication activities can make them more strategic and effective (Besley et al., 2019), and intentionality is needed for science communication to be inclusive (Canfield et al., 2020). The PB-ISC, specifically the behavioral intent factor, could be used before and after a training focused on the importance of planning science communication activities in order to evaluate the efficacy of the training on students’ intentions and planning.
Limitations
We collected validity evidence for the PB-ISC using undergraduate STEM students from diverse majors at an R1 institution. Assessing validity of these indices for students at other institution types would be valuable. Additionally, gathering larger datasets from more diverse institution types would enable analysis for measurement invariance across multiple demographics that are relevant to science communication, such as race (Rodrigues et al., 2023) or gender (Lewenstein, 2019; Rasekoala, 2019). Although there was configural invariance, we did not achieve metric invariance. Measurement noninvariance could indicate important findings about how students of different groups think about inclusive science communication, which could also be a valuable finding (Cieciuch et al., 2019). Future collection of PB-ISC responses from larger samples of students with diverse identities would enable analysis of presence or absence of measurement invariance and facilitate further examination of student perceptions of inclusive science communication.
Some limitations of our data indicate a high skew on the beliefs/attitudes/norms scale, and a slight skew on the self-efficacy scale. However, this is expected based on students’ overconfidence in their beliefs and skills compared with their actual ability to perform a behavior; such Dunning–Kruger effect has been shown in regards to communication-related skills like information literacy (Mahmood, 2016). These are common struggles for students with self-assessment surveys in general (Dunning et al., 2004; Brown et al., 2015), rather than the PB-ISC in particular.
Our cognitive interviews, while limited to n = 5 in number, indicated some important considerations for utilization of the PB-ISC scale. Some students mentioned that the term “socioscientific issue” is vague, although they were able to define the term. In future studies, it would be valuable to test administration of the survey with “socioscientific issue” replaced with something specific that students are considering in their major, such as climate change or vaccines; there is precedent for making a survey specifically referencing student fields of study (Alam et al., 2023).
Implications for Biology Education Researchers and Practitioners
What are our goals of science communication education? Is it just helping students report their Western science findings to “nonscientist” audiences? Or can our science communication training help students consider new perspectives in how they do science? We support the claim that the culture of science communication education needs to be more justice-oriented (Judd and McKinnon, 2021), and that measuring how students are considering communication within and beyond scientific communities is critical. The PB-ISC can help us as researchers and practitioners assess the efficacy of science communication training, which is currently lacking in the field (Vickery et al., 2023). Additionally, this tool can help researchers and practitioners assess the efficacy of related instruction or experiences in adjacent topics that have been shown to support students’ moral reasoning and scientific argumentation, like socioscientific issues (Zeidler and Nichols, 2009; Sadler et al., 2017; Romine et al., 2020; Owens et al., 2022; Klaver et al., 2023), citizen science (Bonney et al., 2014; Phillips et al., 2019; Roche et al., 2020), and science civic engagement (Garibay, 2015; Labov et al., 2019; Dauer et al., 2021; Alam et al., 2023). Finally, the PB-ISC could be used longitudinally once measurement invariance is established across timepoints, to assess students’ growth in their inclusive science communication mindsets and skillsets in response to diverse training or experiences in science communication and community engagement. Additionally, longitudinal tracking could help assess whether increases in students “intents” factor leads to increases in their “behaviors” factor, which would help assess how science communication intentions lead to science communication behaviors.
The Importance of Self-Assessment Surveys in Inclusive Science Communication Education
In addition to the utility of a survey scale for summative evaluation and research purposes, a self-assessment survey can be helpful for student formative assessment and feedback as well as the opportunity to practice reflexivity. While some students may perceive that self-assessment surveys lack utility (Yan et al., 2023), encouraging students to recognize the value of self-assessment is critical. Self-assessment can be formative in helping students evaluate their own perspectives, strengths, and weaknesses in inclusive science communication (Andrade, 2019). Reflexivity challenges people to consider how their identities and goals influence their research and actions, and is an especially important tenet in science communication (Chilvers, 2013; Salmon et al., 2014; Canfield et al., 2020; Jensen, 2022), and self-assessment is an important tool for reflexivity. Guiding students in the importance of self-assessment can increase their reflection abilities (Kangaslampi et al., 2022). Additionally, using the PB-ISC to help students assess their own behavioral intentions in science communication could also help students recognize any lack of intention or planning in their science communication and increase this vital skill (Besley et al., 2019). Coupling PB-ISC self-assessment data with other metrics on students’ actual behaviors in science communication, such as what is included in science communication products that students create (Shivni et al., 2021), could also provide helpful insight to both instructors/researchers as well as students themselves on how accurate their self-assessment was.
The goal of inclusive science communication education is to help students focus on their own assets as well as value the assets of others for collaborating to cocreate solutions to socioscientific issues. Utilizing the PB-ISC items as a self-reflection exercise could be powerful for improving students’ mindsets and skillsets in inclusive science communication. Social Cognitive Theory (Bandura, 1986) highlights the importance of an individual's environment and social ecology in learning. Callwood and colleagues have highlighted the importance of practicing inclusive science communication at multiple levels of influence and groupings in order to help combat exclusionary cultures in these spaces (Callwood et al., 2022). By focusing students’ self-reflection on their current and future uses of inclusive science communication in relationships and in society, the PB-ISC helps students consider their attitudes, norms, and self-efficacy in order to plan behaviors in inclusive science communication. Overall, helping students grow in these TPB constructs can help students practice inclusive science communication at multiple levels of influence in their lives.
ACKNOWLEDGMENTS
This study was supported by National Science Foundation grant #2225095 to Nicole Kelp.
REFERENCES
- 1991). The theory of planned behavior. Organizational Behavior and Human Decision Processes, 50(2), 179–211. https://doi.org/10.1016/0749-5978(91)90020-T Google Scholar (
- 2002). Perceived behavioral control, self-efficacy, locus of control, and the theory of planned behavior. Journal of Applied Social Psychology, 32(4), 665–683. Google Scholar (
- 2021). Science communication training as information seeking and processing: A theoretical approach to training early-career scientists. Journal of Science Communication, 20(5), A06. https://doi.org/10.22323/2.20050206 Google Scholar (
- 2023). Predictors of Scientific Civic Engagement (PSCE) survey: A multidimensional instrument to measure undergraduates’ attitudes, knowledge, and intention to engage with the community using their science skills. CBE—Life Sciences Education, 22(1), ar3. https://doi.org/10.1187/cbe.22-02-0032 Medline, Google Scholar (
- 2023). Inclusive Science Communication training for first-year STEM students promotes their identity and self-efficacy as scientists and science communicators. Frontiers in Education, 8, 1173661. https://doi.org/10.3389/feduc.2023.1173661. Google Scholar (
American Educational Research Association, American Psychological Association, & National Council on Measurement in Education . (2014). Standards for Educational and Psychological Testing. American Educational Research Association. Google Scholar- 2019). A critical review of research on student self-assessment. Frontiers in Education, 4, 87. https://doi.org/10.3389/feduc.2019.00087 Google Scholar (
- 1986). The explanatory and predictive scope of self-efficacy theory. Journal of Social and Clinical Psychology, 4(3), 359–373. https://doi.org/10.1521/jscp.1986.4.3.359 Google Scholar (
- 1997). Self-efficacy: The Exercise of Control (pp. ix, 604). New York: W H Freeman/Times Books/Henry Holt & Co. Google Scholar (
- 1951). The effect of standardization on a χ2 approximation of factor analysis. Biometrika, 38(3–4), 337–344. https://doi.org/10.1093/biomet/38.3-4.337 Google Scholar (
- 2021). A new normal: Integrating lived experience into scientific data syntheses. Frontiers in Psychiatry, 12, 763005. https://doi.org/10.3389/fpsyt.2021.763005 Medline, Google Scholar (
- 2009). Evolution of co-management: Role of knowledge generation, bridging organizations and social learning. Journal of Environmental Management, 90(5), 1692–1702. https://doi.org/10.1016/j.jenvman.2008.12.001 Medline, Google Scholar (
- 2000). Rediscovery of traditional ecological knowledge as adaptive management. Ecological Applications, 10(5), 1251–1262. Google Scholar (
- 2024). Ecologists prioritize listening to community perspectives when they see the benefit: Norms and self-efficacy beliefs appear to have little impact. Science Communication, 46(4), 511–537. doi/10.1177/10755470241239940 Google Scholar (
- 2022). Strategic communication as planned behavior for science and risk communication: A theory-based approach to studying communicator choice. Risk Analysis, 42(11), 2584–2592. https://doi.org/10.1111/risa.14029 Medline, Google Scholar (
- 2016). Qualitative interviews with science communication trainers about communication objectives and goals. Science Communication, 38(3), 356–381. https://doi.org/10.1177/1075547016645640 Google Scholar (
- 2021). American scientists’ willingness to use different communication tactics. Science Communication, 43(4), 486–507. https://doi.org/10.1177/10755470211011159 Google Scholar (
- 2019). Strategic science communication as planned behavior: Understanding scientists’ willingness to choose specific tactics. PLoS One, 14(10), e0224039. https://doi.org/10.1371/journal.pone.0224039 Medline, Google Scholar (
- 2011). What science communication scholars think about training scientists to communicate. Science Communication, 33(2), 239–263. https://doi.org/10.1177/1075547010386972 Google Scholar (
- 2014). Citizen science. Next steps for citizen science. Science, 343(6178), 1436–1437. https://doi.org/10.1126/science.1251554 Medline, Google Scholar (
- 2015). Accuracy in student self-assessment: Directions and cautions for research. Assessment in Education: Principles, Policy & Practice, 22(4), 444–457. https://doi.org/10.1080/0969594X.2014.996523 Google Scholar (
- 2022). Acknowledging and supplanting white supremacy culture in science communication and STEM: The role of science communication trainers. Frontiers in Communication, 7, 787750. https://doi.org/10.3389/fcomm.2022.787750. Google Scholar (
- 2020). The role of scientific communication in predicting science identity and research career intention. PLoS One, 15(2), e0228197. https://doi.org/10.1371/journal.pone.0228197 Medline, Google Scholar (
- 2020). Science communication demands a critical approach that centers inclusion, equity, and intersectionality. Frontiers in Communication, 5, 2. doi: 10.3389/fcomm.2020.00002 Google Scholar (
- 2022). Motivation in reading primary scientific literature: A questionnaire to assess student purpose and efficacy in reading disciplinary literature. International Journal of Science Education, 44(8), 1230–1250. https://doi.org/10.1080/09500693.2022.2073482 Google Scholar (
- 2013). Reflexive engagement? Actors, learning, and reflexivity in public dialogue on science and technology. Science Communication, 35(3), 283–310. https://doi.org/10.1177/1075547012454598 Google Scholar (
- 2023). Scientists’ deficit perception of the public impedes their behavioral intentions to correct misinformation. PLoS One, 18(8), e0287870. https://doi.org/10.1371/journal.pone.0287870 Medline, Google Scholar (
- 2019). How to obtain comparable measures for cross-national comparisons. KZfSS Kölner Zeitschrift Für Soziologie Und Sozialpsychologie, 71(1), 157–186. https://doi.org/10.1007/s11577-019-00598-7 Google Scholar (
- 2022). Assessing how students value learning communication skills in an undergraduate anatomy and physiology course. Anatomical Sciences Education, 15(6), 1032–1044. https://doi.org/10.1002/ase.2144 Medline, Google Scholar (
- 2022). Understanding the intention-behavior gap: The role of intention strength. Frontiers in Psychology, 13, 923464. https://doi.org/10.3389/fpsyg.2022.923464 Medline, Google Scholar (
- 2020). Contribution of training to scientists’ public engagement intentions: A test of indirect relationships using parallel multiple mediation. Science Communication, 42(4), 508–537. https://doi.org/10.1177/1075547020943594 Google Scholar (
- 2022). Exploring undergraduate biology students’ science communication about COVID-19. Frontiers in Education, 7, 859945. https://doi.org/10.3389/feduc.2022.859945 Google Scholar (
- 2021). Students’ civic engagement self-efficacy varies across socioscientific issues contexts. Frontiers in Education, 6, 628784. https://doi.org/10.3389/feduc.2021.628784 Google Scholar (
- 2004). Flawed self-assessment: Implications for health, education, and the workplace. Psychological Science in the Public Interest, 5(3), 69–106. https://doi.org/10.1111/j.1529-1006.2004.00018.x Medline, Google Scholar (
- 2011). Toward a model of social influence that explains minority student integration into the scientific community. Journal of Educational Psychology, 103(1), 206–222. https://doi.org/10.1037/a0020743 Medline, Google Scholar (
- 2003). The expectancy-value muddle in the theory of planned behaviour—And some proposed solutions. British Journal of Health Psychology, 8(Pt 1), 37–55. https://doi.org/10.1348/135910703762879192 Medline, Google Scholar (
- 2015). STEM students’ social agency and views on working for social change: Are STEM disciplines developing socially and civically responsible students? Journal of Research in Science Teaching, 52(5), 610–632. https://doi.org/10.1002/tea.21203 Google Scholar (
- 1945). A basis for analyzing test-retest reliability. Psychometrika, 10, 255–282. https://doi.org/10.1007/BF02288892 Medline, Google Scholar (
- 2015). Measuring networking as an outcome variable in undergraduate research experiences. CBE Life Sciences Education, 14(4), ar38. https://doi.org/10.1187/cbe.15-03-0061 Link, Google Scholar (
- 2022). Developing open, reflexive and socially responsible science communication research and practice. Journal of Science Communication, 21(4), C04. https://doi.org/10.22323/2.21040304 Google Scholar (
- 2021). A systematic map of inclusion, equity and diversity in science communication research: Do we practice what we preach? Frontiers in Communication, 6, 744365. https://doi.org/10.3389/fcomm.2021.744365 Google Scholar (
- 1974). An index of factorial simplicity. Psychometrika, 39(1), 31–36. https://doi.org/10.1007/BF02291575 Google Scholar (
- 1992). An argument-based approach to validity. Psychological Bulletin, 112(3), 527–535. https://doi.org/10.1037/0033-2909.112.3.527 Google Scholar (
- 2022). Students’ perceptions of self-assessment and their approaches to learning in university mathematics. LUMAT: International Journal on Math, Science and Technology Education, 10(1). https://doi.org/10.31129/LUMAT.10.1.1604 Google Scholar (
- 2023). Students’ engagement with Socioscientific issues: Use of sources of knowledge and attitudes. Journal of Research in Science Teaching, 60(5), 1125–1161. https://doi.org/10.1002/tea.21828 Google Scholar (
- 2016). Principles and Practice of Structural Equation Modeling (4th ed). NYC, New York: Guilford Press. Google Scholar (
- 2020). Measuring university students’ interest in biology: Evaluation of an instrument targeting Hidi and Renninger's individual interest. International Journal of STEM Education, 7(1), 23. https://doi.org/10.1186/s40594-020-00217-4 Google Scholar (
- 2019). One size doesn't fit all: Using factor analysis to gather validity evidence when using surveys in your research. CBE Life Sciences Education, 18(1), rm1. https://doi.org/10.1187/cbe.18-04-0064 Link, Google Scholar (
- 2020). Control interactions in the theory of planned behavior: Rethinking the role of subjective norm. Europe's Journal of Psychology, 16(3), 401–417. https://doi.org/10.5964/ejop.v16i3.2056 Medline, Google Scholar (
- 2019). Integrating undergraduate research in STEM with civic engagement. Science Education and Civic Engagement: An International Journal, 11, 1. Retrieved September 25, 2024, from https://files.eric.ed.gov/fulltext/EJ1388822.pdf. Google Scholar (
- 2019). The need for feminist approaches to science communication. Journal of Science Communication, 18(4), C01. https://doi.org/10.22323/2.18040301 Google Scholar (
- 2016). Do people overestimate their information literacy skills? A systematic review of empirical evidence on the Dunning-Kruger effect. Comminfolit, 10(2), 199. https://doi.org/10.15760/comminfolit.2016.10.2.24 Google Scholar (
- 1995). Validity of psychological assessment: Validation of inferences from persons’ responses and performances as scientific inquiry into score meaning. American Psychologist, 50(9), 741–749. https://doi.org/10.1037/0003-066X.50.9.741 Google Scholar (
- 2022). Participatory science communication for transformation. Journal of Science Communication, 21(2), E. https://doi.org/10.22323/2.21020501 Google Scholar (
- 2013). Introducing dyadic interviews as a method for collecting qualitative data. Qualitative Health Research, 23(9), 1276–1284. Medline, Google Scholar (
- 2023). Undergraduate STEM students’ science communication skills, science identity, and science self-efficacy influence their motivations and behaviors in STEM community engagement. Journal of Microbiology & Biology Education, 24, e00182–22. https://doi.org/10.1128/jmbe.00182-22 Medline, Google Scholar (
- 2019). Beyond the deficit model: The ambassador approach to public engagement. BioScience, 69(4), 305–313. https://doi.org/10.1093/biosci/biz018 Google Scholar (
National Academies of Sciences, Engineering, and Medicine; Division of Behavioral and Social Sciences and Education; Board on Behavioral, Cognitive, and Sensory Sciences; Committee on Advancing Antiracism, Diversity, Equity, and Inclusion in STEM Organizations ; (eds.) (2023). Lived experiences and other ways of knowing in STEMM. Advancing Antiracism, Diversity, Equity, and Inclusion in STEMM Organizations: Beyond Broadening Participation. Washington, DC: National Academies Press. Retrieved September 25, 2024, from https://www.ncbi.nlm.nih.gov/books/NBK593029/ Google Scholar- 2021). Conducting research in a post-normal paradigm: Practical guidance for applying co-production of knowledge. Frontiers in Environmental Science, 9, 337. https://doi.org/10.3389/fenvs.2021.699397 Google Scholar (
- 2015). The effectiveness of community engagement in public health interventions for disadvantaged groups: A meta-analysis. BMC Public Health, 15(1), 129. https://doi.org/10.1186/s12889-015-1352-y Medline, Google Scholar (
- 2022). Exploring undergraduates’ breadth of socio-scientific reasoning through domains of knowledge. Research in Science Education, 52(6), 1643–1658. https://doi.org/10.1007/s11165-021-10014-w Google Scholar (
- 2017). Situating interventions to bridge the intention–behaviour gap: A framework for recruiting nonconscious processes for behaviour change. Social and Personality Psychology Compass, 11(7), e12323. https://doi.org/10.1111/spc3.12323 Google Scholar (
- 2023). (Mis)Alignment of challenges and strategies in promoting inclusive racial climates in STEM graduate departments. AERA Open, 9. https://doi.org/10.1177/23328584231168639 Google Scholar (
- 2019). Engagement in science through citizen science: Moving beyond data collection—Phillips—2019—Science Education—Wiley Online Library. Science Education, 103(3), 665–690. Google Scholar (
- 2016). Measurement invariance conventions and reporting: The state of the art and future directions for psychological research. Developmental Review : DR, 41, 71–90. https://doi.org/10.1016/j.dr.2016.06.004 Medline, Google Scholar (
R Core Team (2023). R: A language and environment for statistical computing. Vienna, Austria. Google Scholar- 2019). The seeming paradox of the need for a feminist agenda for science communication and the notion of science communication as a ‘ghetto’ of women's over-representation: Perspectives, interrogations and nuances from the global south. Journal of Science Communication, 18(4), C07. https://doi.org/10.22323/2.18040307 Google Scholar (
- 2016). Contemporary test validity in theory and practice: A primer for discipline-based education researchers. CBE—Life Sciences Education, 15(1), rm1. https://doi.org/10.1187/cbe.15-08-0183 Link, Google Scholar (
- 2022). Motivations and barriers for young scientists to engage with society: Perspectives from South Africa. International Journal of Science Education, Part B, 12(2), 157–173. https://doi.org/10.1080/21548455.2022.2049392 Google Scholar (
- 2020). Addressing diversity and inclusion through group comparisons: A primer on measurement invariance testing. Chemistry Education Research and Practice, 21(3), 969–988. https://doi.org/10.1039/D0RP00025F Google Scholar (
- 2020). Citizen science, education, and learning: Challenges and opportunities. Frontiers in Sociology, 5. Retrieved from https://www.frontiersin.org/articles/10.3389/fsoc.2020.613814 Medline, Google Scholar , ... & (
- 2020). A scale to measure science communication training effectiveness. Science Communication, 42(1), 90–111. https://doi.org/10.1177/1075547020903057 Google Scholar (
- 2023). Minoritized scientists in the United States: An identity perspective to science communication. Science Communication, 45(5), 567–595. https://doi.org/10.1177/10755470231199955 Google Scholar (
- 2020). Measurement of socio-scientific reasoning (SSR) and exploration of SSR as a progression of competencies. International Journal of Science Education, 42(18), 2981–3002. https://doi.org/10.1080/09500693.2020.1849853 Google Scholar (
- 2012). Improving survey methods with cognitive interviews in small- and medium-scale evaluations. American Journal of Evaluation, 33(3), 414–430. https://doi.org/10.1177/1098214012441499 Google Scholar (
- 2007). What do students gain by engaging in socioscientific inquiry? Research in Science Education, 37, 371–391. Google Scholar (
- 2017). Evolution of a model for socio-scientific issue teaching and learning. International Journal of Education in Mathematics, Science and Technology, 5(2), 75–87. Google Scholar (
- 2014). The Reflexive Scientist: Enabling more effective science communication and public engagement through deeper reflection and engagement between physical and social scientists. PA11C-3882. American Geophysical Union, Fall Meeting Abstracts. Google Scholar (
- 2022). Talking science: Undergraduates’ everyday conversations as acts of boundary spanning that connect science to local communities. CBE Life Sciences Education, 21(1), ar12. https://doi.org/10.1187/cbe.21-06-0151 Medline, Google Scholar (
- 2016). The intention–behavior gap. Social and Personality Psychology Compass, 10(9), 503–518. https://doi.org/10.1111/spc3.12265 Google Scholar (
- 2021). Establishing a baseline of science communication skills in an undergraduate environmental science course. International Journal of STEM Education, 8(1), 47. https://doi.org/10.1186/s40594-021-00304-0 Medline, Google Scholar (
- 2016). The lure of rationality: Why does the deficit model persist in science communication? Public Understanding of Science, 25(4), 400–414. https://doi.org/10.1177/0963662516629749 Medline, Google Scholar (
- 2012). Future work selves: How salient hoped-for identities motivate proactive career behaviors. Applied Psychology, 97(3), 580–598. Google Scholar (
- 2016). In science communication, why does the idea of the public deficit always return? Exploring key influences. Public Understanding of Science, 25(4), 415–426. https://doi.org/10.1177/0963662516629750 Medline, Google Scholar (
- 2018). The information deficit model and climate change communication. The Oxford Encyclopedia of Climate Change Communication. New York, NY: Oxford University Press. Retrieved September 25, 2024, from http://www.oxfordreference.com/view/10.1093/acref/9780190498986.001.0001/acref-9780190498986-e-301 Google Scholar (
- 2020). Multiple-group invariance with categorical outcomes using updated guidelines: An illustration using Mplus and the lavaan/semTools packages. Structural Equation Modeling: A Multidisciplinary Journal, 27(1), 111–130. https://doi.org/10.1080/10705511.2019.1602776 Google Scholar (
- 2008). Towards an analytical framework of science communication models. In D. ChengM. ClaessensT. GascoigneJ. MetcalfeB. SchieleS. Shi (Eds.), Communicating Science in Social Contexts: New Models, New Practices (pp. 119–135). Netherlands: Springer. https://doi.org/10.1007/978-1-4020-8598-7_7 Google Scholar (
- 2000). A review and synthesis of the measurement invariance literature: Suggestions, practices, and recommendations for organizational research. Organizational Research Methods, 3(1), 4–70. https://doi.org/10.1177/109442810031002 Google Scholar (
- 2023). Lived experience experts: A name created by us for us. Expert Review of Hematology, 16(sup1), 7–11. https://doi.org/10.1080/17474086.2023.2178410 Medline, Google Scholar (
- 2023). Analysis of inclusivity of published science communication curricula for scientists and STEM students. CBE—Life Sciences Education, 22(1), ar8. https://doi.org/10.1187/cbe.22-03-0040 Medline, Google Scholar (
- 2021). A framework & lesson to engage biology students in communicating science with nonexperts. The American Biology Teacher, 83(1), 17–25. https://doi.org/10.1525/abt.2021.83.1.17 Google Scholar (
- 2018). Exploratory factor analysis: A guide to best practice. Journal of Black Psychology, 44(3), 219–246. https://doi.org/10.1177/0095798418771807 Google Scholar (
- 2006). Does changing behavioral intentions engender behavior change? A meta-analysis of the experimental evidence. Psychological Bulletin, 132(2), 249–268. https://doi.org/10.1037/0033-2909.132.2.249 Medline, Google Scholar (
- 2013). What do our respondents think we're asking? Using cognitive interviewing to improve medical education surveys. Journal of Graduate Medical Education, 5(3), 353–356. https://doi.org/10.4300/JGME-D-13-00154.1 Medline, Google Scholar (
- 2013). Sample size requirements for structural equation models: An evaluation of power, bias, and solution propriety. Educational and Psychological Measurement, 73(6), 913–934. Google Scholar (
- 2023). A systematic review on students’ perceptions of self-assessment: Usefulness and factors influencing implementation. Educational Psychology Review, 35(3), 81. https://doi.org/10.1007/s10648-023-09799-1 Google Scholar (
- 2005). Whose culture has capital? A critical race theory discussion of community cultural wealth. Race Ethnicity and Education, 8(1), 69–91. https://doi.org/10.1080/1361332052000341006 Google Scholar (
- 2009). Socioscientific issues: Theory and practice. Journal of Elementary Science Education, 21(2), 49–58. https://doi.org/10.1007/BF03173684 Google Scholar (