Who is Represented in the Research on Undergraduate Research Experiences in the Natural Sciences? A Review of Literature
Abstract
Positive outcomes from undergraduate research experiences (UREs) have resulted in calls to broaden and diversify participation in research. However, we have little understanding of what demographics are reported and considered in the analyses of student outcomes from UREs. Without this information, it is impossible to assess whether participation in UREs has been diversified and how outcomes may vary by participant identity. Through a comprehensive literature search, we systematically identified 147 peer-reviewed research articles on student participation in UREs in the natural sciences, published between 2014 and 2020. We coded each paper to document which student demographic variables are reported and considered in analyses. The majority (88%) of articles on UREs reported at least one demographic variable and 62% incorporate demographics into their analyses, but demographics beyond gender and race/ethnicity were infrequently considered. Articles on independent research apprenticeships included demographics in their analyses more frequently than studies on course-based undergraduate research experiences (CUREs). Trends in reporting and analyzing demographics did not change from 2014 to 2020. Future efforts to collect these data will help assess whether goals to diversify UREs are being met and inform how to design UREs to meet the needs of diverse student groups.
INTRODUCTION
“[Undergraduate research experiences] have been proposed as an opportune way to actively engage students and may be a key strategy for broadening participation in [Science, Technology, Engineering, and Math (STEM) fields](…) Current efforts are working to increase the number of students participating in undergraduate research experiences and to increase the diversity of those participants.”—National Academies report, Undergraduate Research Experiences for STEM students, pg. 3
The scientific community does not mirror the demographics of society (National Science Foundation, 2019). For far too long, the scientific community has been dominated by white, cisgender, straight, able-bodied, privileged men who have created a culture of science in which others have found it difficult to thrive (Malcom et al., 2016; Asai, 2020). Many studies have taken a student-deficit approach to explain why we see underrepresentation in science (Castro, 2014), but a growing body of evidence is contradicting the longstanding deficit narrative (Harper, 2010) that women, people of color, or people from low socioeconomic backgrounds are simply not interested in science (Hughes, 2018) or are unprepared for the rigor of university science (Byars-Winston et al., 2016). Rather, there are systemic challenges that impact persistence in undergraduate science education (Seymour and Hunter, 2019) for students from underserved racial and ethnic groups (Estrada et al., 2016), women (Eddy and Brownell, 2016), LGBTQ+ students (Hughes, 2018; Maloy et al., 2022), and low socioeconomic status students (MacPhee et al., 2013). Studies have pointed to a myriad of factors that influence student persistence in science, including a low sense of belonging and feelings of isolation (Rainey et al., 2018), faculty instructors who are not approachable or are not perceived to care about students (Zydney et al., 2002; Rainey et al., 2019), few role models and mentors in science with similar identities (Pfund, 2016), research anxiety (Cooper et al., 2023), and systemic issues of racism (Bonilla, 2006; Edge, 2020; Makgoba, 2020; McGee, 2020), sexism (Clancy et al., 2014; Hughes et al., 2017; Kuchynka et al., 2018), homophobia (Cech and Waidzunas, 2021), and classism in science (Garriott et al., 2021).
In recent years, institutional change efforts to address inequities and prevent the attrition of traditionally underserved students in undergraduate sciences have featured calls for increased student involvement in undergraduate research experiences (UREs) (Brewer and Smith, 2011; PCAST, 2012). Traditionally, UREs are independent experiences taking place in faculty research groups. Undergraduate research has been hailed as a universally beneficial component of undergraduate STEM education (Russell et al., 2007) and a high impact practice that can increase persistence in STEM (Eagan et al., 2013; Graham et al., 2013). With the limited number of independent UREs available in faculty member research groups, the development and expansion of course-based undergraduate research experiences (CUREs) have enabled these research experiences to be embedded into formal courses, theoretically making research accessible to a far greater number of students and broadening opportunities to more diverse student groups (Bangera and Brownell, 2014).
However, it is not clear who is represented in the current literature that demonstrates the benefits of UREs and whether these benefits are equally distributed to students with underserved identities. Notably, researchers have critiqued extrapolating extensively from the studies that provide evidence for the benefits of UREs because this body of literature includes few randomized controlled studies, few multi-institution studies, and self-selection bias that may overrepresent individuals from privileged backgrounds (Linn et al., 2015; National Academies of Sciences, Engineering, and Medicine, 2017; Cooper et al., 2021). However, a more basic question is what student demographics are represented in the extant literature that evaluates outcomes of undergraduate research. Knowing who is represented in the literature can help institutions assess whether studies may be applicable to their demographic contexts or whether certain demographic groups may have differential experiences garnering benefits.
In this work, we use the term “underserved” to refer to any group that may be systematically underprivileged in traditional university settings. We intentionally avoid using the term “underrepresented,” because some may use “underrepresented” to specifically refer to racial/ethnic underrepresentation, while others may use this term to describe any group with numbers lower than the proportional representative in the general population. We do not restrict the “underserved” term to race/ethnicity, but also consider how universities were not originally designed to serve a number of student identities—including students of color, women, LGBTQ+ students, students with disabilities, first-generation students, low-income students, nontraditionally aged students, and more. In this work, we do not attempt to report specific outcomes for these groups. Rather, we aim to simply understand whether the literature on UREs is reporting on these demographic variables and considering these variables in their study analyses, which would enable a broader understanding of the specific experiences of students with these identities in UREs.
There is evidence for demographic differences potentially impacting students’ decisions to persist in their research experiences. For example, first-generation students are more likely to persist in research experiences due to financial benefits, while continuing-generation students are more likely to persist because they perceive they are gaining important skills and knowledge (Cooper et al., 2019). The motivation of personally valuing a task (e.g., recognizing that one is gaining important skills and knowledge from their research experiences) is linked to student persistence, while external motivators (such as financial benefits) are linked to decreased well-being (Howard et al., 2021). If student identity can impact motivation to enter and persist in an URE, we might expect differences in the academic and affective outcomes that students with different identities experience due to participation in undergraduate research.
To date, there is very little in the research literature to tell us how UREs may differentially impact students with different identities, which can result in an erroneous monolithic interpretation of student outcomes from UREs (for exceptions, see Aikens et al., 2017; Cooper et al., 2019; Gin et al., 2021). Given the national calls for universities to incorporate more research into their undergraduate curriculum (Brewer and Smith, 2011; PCAST, 2012), strong generalizations about the positive impacts of undergraduate research likely have already impacted policy and funding decisions at many institutions. However, we may need to take a step back to consider whether the positive impacts of participating in undergraduate research are true for students from underserved backgrounds. Perhaps UREs by design work best to increase the persistence of students from majority groups who are already privileged in academia and may be less impactful for underserved students. Failure to understand whether we are appropriately supporting underserved students through research experiences has negative impacts not only for the students themselves, but also for the advancement of science: underserved students bring unique affordances into research, and new perspectives and thinking which can help us counteract bias and lead to better scientific research (Intemann, 2009). For example, students with disabilities highlight ways that their disability identity strengthens their capacity and ability to contribute to science, including by contributing to students’ resilience, creativity and problem-solving skills, and ability to hyperfocus (Gin et al., 2022). We therefore need to look to the existing research literature to understand the degree to which students of different backgrounds and identities are represented in the current literature, so we can make informed decisions about how UREs may contribute to diversity, equity, and inclusion efforts in undergraduate education.
To address this question of who is represented in the research on UREs in the natural sciences, we examined to what extent student identities have been reported and considered in analyses in the research literature on student outcomes from participation in UREs. Because of the growing popularity of CUREs as a mechanism to broaden participation in research, we include both CUREs and independent UREs in faculty member labs. For clarity throughout this paper, the term “UREs” refers to all research experiences—including CUREs and independent research experiences. The term “independent UREs” refers specifically to independent research experiences conducted in faculty members’ research groups (this is also sometimes referred to as research apprenticeships). We hypothesize that the methods used to evaluate student outcomes in each study could influence the extent to which demographic data are reported and considered in analyses. Further, we expect that trends of considering demographic variables in analyses would have increased over time, alongside increased attention toward diversity, equity, and inclusion in undergraduate education. Our specific research questions are:
To what extent are student demographic groups reported and considered in analyses in studies focused on UREs?
Are there differences in the extent to which student demographics groups are considered in analyses between studies with data from research in independent UREs compared with CUREs?
Are there differences in the extent to which student demographic groups are considered in analyses between studies with primarily quantitative, primarily qualitative, or mixed methods data?
Are there differences in the extent to which student demographic groups have been reported and considered in analyses over time?
MATERIALS AND METHODS
Positionality
This study was conducted as part of a CURE for community college transfer students at a research-intensive institution. The research project spanned the Fall 2020 and Spring 2021 semesters and the team consisted of 17 undergraduate students, a graduate student (L.E.G.), a postdoctoral scholar (E.C.G.), and two professors (K.M.C. and S.E.B.). All researchers had personal experience as an undergraduate researcher in independent UREs, as well as experience either as a student or as an instructor of CUREs. Many of the researchers hold identities considered in the current study, including identifying as: men, women; white, Black, Latinx, Indigenous; LGBTQ+; nontraditionally-aged students; having mental health conditions such as depression or anxiety; having disabilities; first-generation college students; having low socioeconomic status; community college transfer students; honors students; balancing employment with college; speaking English as a second language; religious; student commuters; primary caregivers; and out-of-state students.
Establishment of Initial Article Parameters
We aimed to conduct a methodical literature search to identify peer-reviewed articles that evaluated outcomes of participation in UREs in the natural sciences. To focus our analysis, we limited our search to studies published between 2014 and 2020, since the literature on CUREs started growing following the 2014 publication of a meeting report that defined key components of CUREs (Auchincloss et al., 2014). All articles that were included evaluated research experiences in the natural sciences, and we defined eight categories that captured how authors described students’ research experiences in their studies: 1) biology, 2) health sciences, 3) biochemistry, 4) chemistry, 5) physics, 6) geosciences, 7) STEM, and 8) STEM + non-STEM. Biology was used to include articles with research experiences in life sciences, microbiology, genetics, physiology, ecology, evolution, biomedical, and environmental science. Studies evaluating research experiences exclusively in technology, engineering, or math fields without any sciences were omitted from our collection. For example, articles including chemistry research experiences would be included, but if an article only included chemical engineering research experiences, it was not included. Studies on research experiences in multiple STEM disciplines, with at least one natural science discipline represented, were categorized as “STEM.” Studies on research experiences in and outside of STEM, with at least one natural science discipline represented, were categorized as “STEM + non-STEM.” A full list of article parameters are included below, with further description and clarifying information included in the Supplemental Materials (S1).
Article Inclusion Criteria
Peer-reviewed education research article published in an academic journal.
Article was published between 2014 and 2020.
Study focus is undergraduate students (not high school or graduate school) who have participated in research experiences (independent or course-based).
Research experiences occurred at least partially in a natural sciences discipline (i.e., biology or chemistry rather than exclusively engineering or math).
Research experiences took place in the United States.
Article includes empirical data on students (including quantitative, qualitative, or mixed methods studies).
Primary purpose of paper is to describe research on student outcomes, rather than to describe a curriculum or program.
Initial Curation of the Article Library
To find as many articles as possible that fit our criteria, the research team engaged in brainstorming sessions and discussions to generate a list of relevant search terms (e.g., “undergraduate research experience,” “URE.” “research apprenticeship,” “course-based undergraduate research experience”). These terms were used alone and combined with terms related to student demographics (e.g., “demographics,” “gender,” “low-income”), and members of the research team were assigned different combinations of search terms to systematically conduct literature searches via Google Scholar (full list of search terms is provided in the Supplemental Materials, S2).
We used Google Sheets to collect and catalog all relevant articles in our article library. Each article entry included information such as the article citation, search terminology used to find the article, and the discipline(s) included in the study. A total of 446 articles were originally identified and cataloged by the team of 17 researchers. To establish a finalized article library that only included articles with our criteria, two researchers were tasked with reviewing all articles in the article library to revise entries when necessary and determine whether any did not fit in the collection (e.g., an essay on CUREs, conference proceedings, a dissertation, etc.). The two researchers discussed the qualifications of each article to consensus, and questionable articles were reviewed by a third researcher. This review process resulted in an initial library of 273 articles.
Development of the Preliminary Coding Rubric and Coding Form
A subset of the research team convened to discuss the study goals and review studies within the article library, and through these discussions drafted a preliminary coding rubric to capture descriptive characteristics of each research article as well as the types of demographics reported and analyzed in each study. Examples of article characteristic items include codes such as “Qualitative Study” and “Study on CUREs,” while demographic items included “Socioeconomic Status Reported” and “Gender Considered in Analysis.”
For a demographic item to count as having been reported, the data had to have been presented in a quantitative form such as numbers, percentages, averages, or sample sizes (e.g., 40% first-generation students). General or vague statements about the presence of a demographic variable (e.g., “there were some women in the class”) were insufficient to be coded as “reported” in our coding scheme. For a demographic item to count as having been considered in analysis, the study had to include statistical, quantitative, or qualitative comparisons between or within demographic groups (e.g., “women were 3x more likely to persist in research than men”). Additionally, studies that interpreted qualitative data through the lens of a specific demographic group were also coded as considering that demographic in their analyses—for example, an interview study exploring the experiences of women of color would be coded as considering both “gender” and “race/ethnicity” in the analyses. Finally, the coding rubric also captured whether demographics were recorded in the main article or in the Supplemental Materials of each paper.
The coding rubric included an in-depth description of each item, instructions on how to code for each item, and examples of what should not be coded for each item. Modeled after the process of Krim et al., (2019), we then transferred the rubric into a user-friendly Google form that all 17 student researchers could use to code and collate article entries (Supplemental Materials, S3). The initial draft of the rubric and coding form was brought before the entire research team for collaborative review and comments, and edits and clarifications were made as needed.
Refinement of the Preliminary Coding Rubric and Coding Form
Researchers were assembled into coding teams of three, and each coding team was assigned sets of 10 articles at a time to code using the preliminary coding rubric. While researchers within the same team coded the same set of articles, they coded independently to reduce bias. Coding each article involved reviewing all parts of the published article and any associated Supplemental Materials, and coding results from each submitted Google form were automatically compiled into a Google sheet that members of the coding teams could not view. After coding a set of 10 articles, the entire research team was able to see the Google sheet and met to discuss discrepancies and issues that came up while coding. The rubric and coding form were then clarified and revised to improve coding accuracy. This process was repeated five times to iteratively refine the coding rubric, and in total, each coding team coded 50 articles in the process of developing the coding rubric. At the end of the fifth session, all researchers were in agreement that the coding rubric and form could be used to effectively code the articles with a high degree of agreement between coders and across coding teams. The final coding rubric and coding form are included in the Supplemental Materials (S1 and S3). The demographic characteristics that we coded for were: gender; race/ethnicity; major; grade-point-average (GPA); generation status; prior research experience; class level (year in school); career goals; standardized testing scores; age; socioeconomic status; number of credits earned; mental health status; honor’s student; employment status; disability status; community college transfer student; English as second language; commuter status; caregiving status; state residency status; religious identity; military status; LGBTQ+ status, and international student status.
Final Article Coding and Review
After the final coding rubric and coding sheet were established, all the articles in the library were evenly distributed among the coding teams. Individuals in each coding team coded the same articles independently, and the results were compared among groups of three. Following the completion of coding, five researchers reviewed all instances where members of a coding team were not unanimous in their coding, and this review team coded all discrepancies to consensus.
Postcoding Curation of the Article Library
During the coding process, researchers identified 17 articles that did not meet the study parameters, and these articles were removed from the library. Following the coding process and prior to data analysis, researchers also determined that two new additional criteria should be necessary for inclusion in our study. First, we decided to only include U.S.-based studies and removed 25 international studies from our library because the UREs were distinctively different from many U.S. experiences (e.g., research experiences for undergraduate medical school students) or because of contextual differences that complicated demographic data collection. Second, the researchers realized that although all the articles in the library included peer-reviewed data on student outcomes in research experiences, many of these articles were primarily focused on describing the implementation of specific curricula or programs (e.g., articles published in the “Curriculum” section of the Journal of Microbiology and Biology Education). These curriculum articles often provide student data to serve as evidence of effectiveness of the specific curriculum described, but the description of the curriculum, rather than rigorous evaluation of student outcomes, is the primary purpose of curriculum studies. In contrast, research articles occasionally described curricula but primarily served to contribute novel information about outcomes from participation in UREs to the audience. We observed that the way student data were collected, analyzed, and shared was often starkly different in curriculum articles as compared with research articles, in that student data were often not systematically collected or analyzed in curriculum articles. Because the current study seeks to understand who is represented in the research literature on UREs, we decided to exclude articles that primarily describe curriculum or programs from our study.
Two researchers independently conducted a final review of articles to screen out articles that did not meet all the criteria described above (Table 1) and discussed any discrepancies to consensus. Of the 231 articles that met all other described parameters, we determined that 84 were primarily curriculum articles, and 147 were primarily research articles, so we continued analysis strictly on the 147 research articles (citations for each research article are provided in Supplemental Materials, S4).
Data Analysis
After establishing the final article library (n = 147), we collected descriptive statistics including frequencies of reporting and considering specific demographic variables in analysis. Pearson’s chi-square tests were used to assess differences in the proportions of studies that report and consider gender and race/ethnicity in their analyses for studies that varied by URE type (independent vs. CURE) and methodological type (quantitative, mixed-method, or qualitative). Chi-square tests were also used to assess differences in the proportions of studies that reported or considered at least one demographic variable in analyses across different study methodological types. One-way analysis of variance (ANOVA) tests were conducted to assess differences in the number of unique demographic variables that were reported or analyzed across different study methodological types.
Simple linear and binomial logistic regression models were conducted to test whether trends in reporting and analyzing demographic variables changed between the years 2014 and 2020. All data analyses and data visualization were conducted in R (R Core Team, 2019; RStudio Team, 2019).
RESULTS
Article Characteristics
Of the 147 research studies in our final article library, 61% of studies focused solely on independent UREs, 36% focused on CUREs, and 3% considered both independent UREs and CUREs. Nearly half (46%) of all studies were focused on UREs in biology, while 32% focused on STEM disciplines broadly and 10% considered both STEM and non-STEM disciplines. The remaining 11% of studies considered other specific scientific disciplines. Three-quarters (74%) of the studies on CUREs took place in biology. The full breakdown of disciplines by type of research experience can be found in Supplemental Materials (S5).
Over half (54%) of the studies in our final article library used solely quantitative methods, while 34% were mixed methods studies employing both quantitative and qualitative methods. Twelve percent of studies used only qualitative methods, and these studies generally considered smaller sample sizes (Figure 1A)—a trend that is expected, given that sample size recommendations for qualitative studies generally range from just 5 to 60 participants (Creswell and Creswell, 2018; Bekele and Ago, 2022). We observed no discernable pattern between sample size and type of research experience (independent UREs, CUREs, or both) considered in each study (Figure 1B).
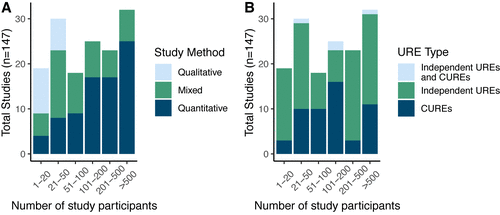
FIGURE 1: Distribution of sample size by (A) study methods and (B) URE type for studies exploring student outcomes on undergraduate research experiences.
Finding #1: Most Research Studies on UREs do not Report or Consider Demographic Characteristics Beyond Gender and Race/Ethnicity
We found that the majority (88%) of research studies on UREs (including both independent research experiences and CUREs) reported at least one demographic variable to describe students in their study. Most (62%) research studies also considered at least one demographic variable in their analyses. On average, research studies reported descriptive information for four different demographic variables and considered two different demographic variables in their analyses.
We calculated the percentage of the 147 studies that reported or considered in analyses each of the demographic variables (Figure 2; Supplemental Materials, S6). While 80% of studies reported information on gender, and 69% of studies reported race/ethnicity data, gender was only considered in analyses for 44% of studies, and race/ethnicity for 42% of studies. All other demographic information was reported and analyzed at significantly lower rates. Academic characteristics of the student, such as GPA, major, or class level were used in analyses for less than 20% of articles. Prior research experience and career goals, which would be predicted to influence student experience and outcomes in either an independent URE or a CURE, were used in the analyses in similarly few articles. Few studies explored the impact of student-level characteristics such as socioeconomic status, mental health, English as a second language, disability status, and whether students are working a job or commuting. Notably, only two studies in our sample considered community college transfer status or caregiving status in their analyses, and zero studies in our sample reported or analyzed information about religious identity, military status, LGBTQ+ identity, or international student status (Figure 2).
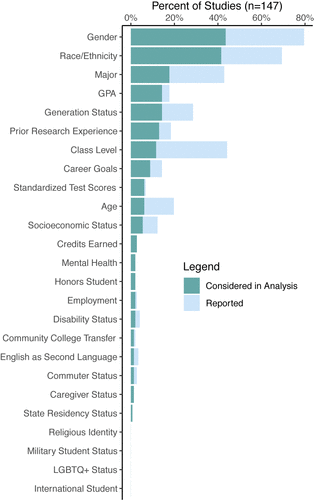
FIGURE 2: Percent of research studies (including both independent UREs and CUREs) that report and consider in analyses each demographic variable in their study. While gender and race/ethnicity are reported and considered in analyses for many studies, other demographic variables are infrequently considered.
Finding #2: Demographic Variables are More Frequently Analyzed in Studies on Independent UREs than in CUREs
We considered whether there were differences in which demographic variables were considered in analyses for studies examining outcomes of independent UREs compared with studies examining outcomes from CUREs (Supplemental Materials, S7). Omitting four studies that included data on both independent UREs and CUREs, we found that studies on independent UREs were significantly more likely to consider any demographic variable in their analyses at all (71% for independent UREs vs. 47% for CUREs; P < 0.01). On average, independent UREs were more likely to both report and consider a higher number of unique demographic variables in their analyses (reported mean = 4.46 [SD = 2.6] variables vs. 3.5 [2.3] for CUREs, P < 0.05; considered 2.6 [2.7] variables vs. 1.3 [1.9] for CUREs, P < 0.01; Supplemental Materials, S8).
Further analyses revealed that studies on independent UREs specifically considered gender and race/ethnicity in their analyses at significantly higher rates than studies on CUREs (Gender: χ2 = 8.78, df = 1, P < 0.01; Race/ethnicity, χ2 = 8.66, df = 1, P < 0.01; Figure 3). Due to small group sizes, we did not calculate chi-square tests to test for statistical differences in the extent to which other demographic variables were considered in analyses between studies on independent UREs and CUREs. However, nearly every demographic variable was more frequently considered in analyses in studies on independent UREs (Figure 3).
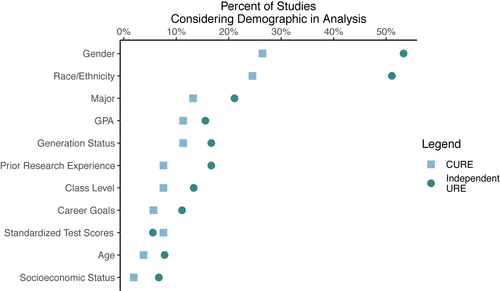
FIGURE 3: Percent of studies on CUREs and independent UREs that consider each demographic in their analyses. Significantly higher proportions of studies on independent UREs (green circles) considered gender and race/ethnicity in their analyses as compared with studies on CUREs (blue squares). Other demographic variables were also more frequently considered in analyses in studies on independent UREs.
Finding #3: Demographics are More Frequently Considered in Analyses in Quantitative Research Studies, Compared with Mixed Methods or Qualitative Studies
We expected that there might be differences in the extent to which demographic variables were reported and analyzed depending on the type of methods used in each study (Supplemental Materials, S9). While we found no significant differences in how demographic variables were reported by methodological type of study (quantitative, mixed methods, or qualitative), quantitative studies on average considered higher numbers of demographic variables in their analyses (quantitative studies mean = 2.7 [SD = 2.8] variables versus mixed methods 1.5 [2.1] versus qualitative 1.3 [1.3]; P < 0.01; Supplemental Materials, S10). Given the nature of quantitative work, and acknowledging that quantitative studies generally have larger sample sizes (Figure 1) that permit for further demographic analyses while maintaining participant confidentiality, it makes sense that quantitative studies consider more demographic variables in their analyses.
We found no significant differences in the percent of quantitative, mixed methods, and qualitative studies that considered gender or race/ethnicity in analyses (Gender: χ2 = 4.89, df = 2, P = 0.09; Race/ethnicity, χ2 = 6.01, df = 2, P = 0.05; Figure 4). Small group sizes prevented us from calculating chi-square tests for differences in the extent to which other demographic variables were considered in analyses.
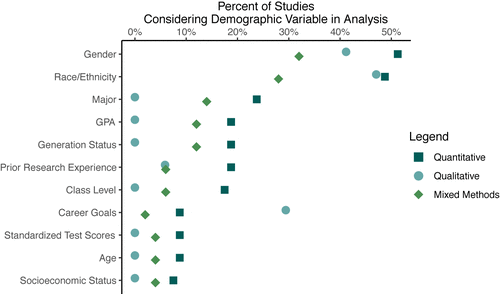
FIGURE 4: Percent of studies on UREs that consider each demographic in their analyses, visualized by methodological type. Quantitative research studies (dark blue squares) tend to consider specific demographic variables in their analyses more frequently than qualitative research studies (light blue circles) or mixed methods studies (green diamonds).
Finding #4: Patterns in Reporting and Considering Demographic Variables in Analysis Have Not Changed Over Time
Regression analyses found no significant trends over time in how studies on UREs treat demographic variables for studies published between 2014 and 2020 (Figure 5; Supplemental Materials, S11). These analyses included a consideration of the number of studies that considered at least one demographic variable in analyses (Figure 5A) and the overall average number of demographic variables each study included in their analyses (Figure 5B).
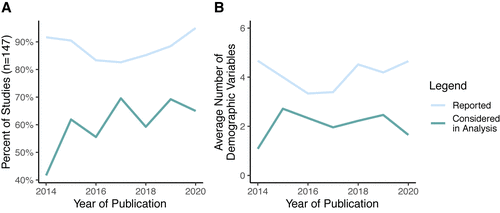
FIGURE 5: Patterns in reporting and considering demographic variables in analysis over time. (A) Between 2014 and 2020, there was no significant trends in whether studies on undergraduate research experiences reported at least one demographic variable or considered at least one demographic variable in their analyses. (B) Between 2014 and 2020, there was no significant change in the average number of demographic variables that studies on undergraduate research experiences either reported or considered in their analyses.
DISCUSSION
This study represents the first to our knowledge to describe the extent to which student demographics are represented in the literature on UREs. In this study, we found that though 62% of the research studies on student outcomes from UREs consider at least one demographic variable in their analyses, demographics beyond gender and race/ethnicity are rarely considered. Patterns in the extent to which demographic variables have been reported and considered in analyses have not changed significantly between 2014 and 2020. Despite calls to broaden participation in undergraduate research based on the positive impacts of undergraduate research on students, we know little about who is represented in the literature and thus, who benefits from these experiences.
Are Calls to Implement UREs to Support Outcomes for Traditionally Underserved Students Supported by the Current Body of Literature?
When we evaluate student outcomes without considering the demographic characteristics of our students, our findings are by default primarily reflecting the outcomes of students in majority groups. According to the 2017 US Census, the majority of undergraduates enrolled at universities across the United States are white, under 25 years old, do not have a disability, and less than half of full-time students are employed (US Census Bureau, 2017). The majority of studies on UREs to date have documented positive outcomes from UREs (National Academies of Sciences, Engineering, and Medicine, 2017). However, without a rigorous understanding of how outcomes vary by different demographic groups, we can only conclude with confidence from the body of research on UREs that these experiences are likely to be beneficial for students who are white, young, do not have a disability, and are not balancing full-time school with employment.
Though we have much less evidence that UREs are effective for students with demographic characteristics and identities that make up minority identities in the population, the positive findings of participation in UREs have resulted in national calls for undergraduate science education reform to engage all science students in UREs (Brewer and Smith, 2011; PCAST, 2012). These calls are made specifically to support outcomes for diverse students who have been traditionally underserved in STEM fields (Brewer and Smith, 2011; PCAST, 2012), and have likely influenced policies and financial decisions at many institutions to invest resources into providing these students with research experiences. Yet, our research indicates that these decisions have not been informed by a large body of evidence supporting that UREs will be particularly beneficial for traditionally underserved students. Further, in the decade since these recommendations were made, we did not observe a significant increase in studies that considered student demographics and identity characteristics in their analyses in studies between 2014 and 2020. If we intend to continue to expand access and involvement in UREs, it is well past time that we expand our knowledge of how different student identities impact outcomes in UREs and we can only do so if we initially collect data on student identity. Further, we need to conduct more research focused on expanding our understanding of how we can develop inclusive UREs to best support students with different identities by seeing whether there are differential experiences.
Is There Evidence for Differential Impacts of Undergraduate Research on Students Based on Identity?
Calls to increase participation in UREs are similar to (and often paired with) calls to increase implementation of active learning practices in undergraduate classrooms. As a field, we have widely accepted the evidence that active learning practices, as an alternative to traditional lecture courses, are beneficial for student learning (Freeman et al., 2014), though we have been less successful in implementing active learning practices across STEM classrooms nationwide (Stains et al., 2018). Active learning has been shown to be disproportionately beneficial at mitigating achievement gaps (Theobald et al., 2020). However, further investigation into how student identities impact interpersonal experiences in active learning classrooms have uncovered differences in student experiences driven by gender, race/ethnicity, political and religious identity, LGBTQ+ identity, commuter status, and disability status (Eddy et al., 2015; Cooper and Brownell, 2016; Henning et al., 2019; Gin et al., 2020; Pfeifer et al., 2023), such that many of these marginalized students face challenges in active learning environments that have to be attended to in order to maximize their experiences in active learning. We can expect that student identities might similarly impact students’ experiences in UREs—and recent work exploring student experiences has supported this.
In the last few years, several studies have highlighted challenges in UREs for students with disabilities and mental health conditions (Braun et al., 2018; Cooper, Gin, Barnes et al., 2020; Cooper, Gin, and Brownell, 2020; Gin et al., 2022; Cooper et al., 2023). Other studies have found that demographic characteristics can impact the tangible scholarly outcomes that students can achieve as part of a research experience. For example, women undergraduate researchers interact less frequently with faculty mentors and achieve lower levels of scholarly productivity (i.e., presenting at conferences, co-authoring manuscripts) as an outcome of their research experiences (Aikens et al., 2017). A lack of participation in these scholarly activities may negatively impact students’ affective outcomes from their research experience, and likely reduce students’ competitiveness for graduate school and careers within STEM fields (Davis and Warfield, 2011).
While both continuing-generation and first-generation students may lack knowledge on how to get involved in UREs, it is likely that first-generation students enter college with less knowledge about how to navigate their college experiences and subsequently may have more difficulty accessing and succeeding in independent research experiences (Collier and Morgan, 2008; Bangera and Brownell, 2014; Cooper et al., 2021). A study of first-generation doctoral students found that compared with continuing-generation students, first-generation students have unstable self-efficacy in their ability to do research, which could impact their persistence and productivity in research (Litson et al., 2021). Continuing-generation undergraduate researchers have increased odds of publishing when they spend more time with their mentors, have increased self-efficacy in research, and have been in research longer—all benefits that are not accrued at the same rates for first-generation undergraduate researchers (Grineski et al., 2018).
Low-income students may also face yet-unexplored barriers in undergraduate research—for example, many faculty members expect undergraduates to begin undergraduate research projects as volunteers or for academic credit, and low-income students are more likely to need to balance these nonpaid research opportunities with the need to have weekly paid employment. Indeed, financial concerns are a known factor for why students consider leaving their UREs—24% of students who leave their research experiences highlight that they needed to spend more time making money (Cooper et al., 2019; Gin et al., 2021). However, we found that only 5.4% of studies on UREs consider socioeconomic status in their analyses, so there may be many more concerns that are currently unknown for these students.
Finally, community college transfer students persist in STEM fields at significantly lower rates than nontransfer students (NSC Research Center, 2017), and involvement in undergraduate research could be a viable mechanism to support persistence of students in STEM fields. However, there are more opportunities for independent faculty-mentored research experiences at 4-year research institutions compared with community colleges, and transfer students have significantly less time to access and benefit from these experiences. Additionally, faculty may preferentially select students who are earlier in their undergraduate careers for research since these students are more likely to be able to contribute to the lab productivity for a longer period of time. In our review, we identified only two studies that considered community college transfer students in their analyses, and only three studies that reported the percentage of transfer students in their sample. This indicates that not only do we know little about outcomes for community college transfer students in research experiences—we also do not know the extent to which community college transfer students participate in research experiences. There have been specific calls to amplify scholarship in biology education research at community colleges, and our findings here support the assertion that additional studies must be focused on community college students and transfer students (Schinske et al., 2017).
Are CUREs an Effective Solution for Supporting Underserved Students in Science?
We were surprised to find that studies on CUREs were almost always less likely to consider specific demographic variables in their analyses (Figure 4). CUREs are often framed as an approach to create more equitable access to research for underserved students (Bangera and Brownell, 2014), yet the lack of CURE studies that consider less-common demographic variables in their analyses indicates that we have little evidence that CUREs are effective in supporting traditionally underserved students. When students are able to choose between participation in a CURE or an independent URE, low-income students and community college transfer students disproportionately participate in CUREs (Shapiro et al., 2015), yet we identified only two studies that considered outcomes of CURE participation for low-income students in their analyses, and only one study that considered community college transfer students. Because independent UREs often require more out-of-class time, we expect that students with additional constraints on their time—such as students who need to commute to campus, are employed, are caregivers, or have military duties, will generally be underrepresented in independent UREs and overrepresented in CUREs. Additional student characteristics, such as being a first-generation student, having a low GPA, having a disability or a mental health condition (e.g., anxiety, depression) (Cooper, Gin, Barnes et al., 2020; Gin et al., 2022), or being a community college transfer student may present specific barriers in access to independent UREs. Consequentially, these students, too, may be more likely to enter CUREs, which do not often require students to apply and be selected for participation, as is often the case for independent UREs. It is therefore critical that we conduct more studies on CUREs to better understand whether, and under what conditions, these experiences support students with these identities. Additionally, while CUREs are prevalent in biology classrooms, they are increasingly being implemented in other scientific disciplines. We found that the majority (74%) of studies on outcomes from CUREs were conducted in biology classrooms (Supplemental Materials, S5), highlighting the need for studies to further explore the efficacy of CUREs for students with different identities in other disciplines.
How Can We Increase Our Understanding of Student Experiences in CUREs and Independent UREs?
In addition to encouraging researchers to directly consider student identity when designing studies on the outcomes of research experiences, we encourage researchers to collect and publish demographic data on participation in UREs even when it is not integral to their research questions or used in analyses. We understand that there are valid reasons why demographic data are sometimes not able to be included—for example, instances where demographic information may compromise participant anonymity in very small qualitative studies, or if so few students in a large quantitative study have a particular identity. However, whenever possible, we encourage education researchers to collect more demographic information in their studies, and to share it in the Supplemental Materials or main text of their work. Even data reported in aggregate to protect participant identity will provide valuable information about the population of students. Anecdotally, we have encountered two central concerns from researchers when we provide this suggestion, which we respond to below:
Concern #1: Collecting demographic questions adds to survey fatigue or makes the survey too long. In our experience, extra demographic questions are very quickly answered with relatively little effort by participants. Making demographic questions optional and asking them at the end of the survey helps ensure that extra demographic questions will not add fatigue that prevents students from responding to the researcher’s main survey items.
Concern #2: Subgroups of people with less common identities will be too small to be informative in data analyses. We recognize that sample size can be a barrier for researchers evaluating outcomes of UREs, because exploring outcomes for small student subpopulations reduces statistical power. Likewise, qualitative researchers likely would feel uncomfortable drawing inferences about experiences with students with identities that are not explicitly discussed by their study participants. However, we encourage researchers to still report out this information even when it cannot be meaningfully incorporated into analyses, so long as the data can be collected and disseminated in a manner that preserves anonymity of the study participants, following the recommendations of the university’s Institutional Review Board. Even when demographic data cannot be used in analyses, including these data in aggregate will broaden our collective understanding of which students are and are not participating in research experiences.
Though many studies will be unable to directly assess the impacts of research experiences for students with identities that are represented in low numbers, it remains important that we seek to understand how we can create supportive and inclusive research environments for these students. Qualitative studies can be critical for identifying what barriers might particularly impact students with less-frequently represented identities, and for informing evidence-based recommendations to develop equitable research experiences.
Limitations
Throughout this study, and particularly throughout our literature search and library curation, we made several intentional decisions that limit the scope of our findings. First, while our systematic literature search was extensive, we may have missed papers that were not indexed on Google Scholar or that used terminology that we did not consider in our search terms (see Supplemental Materials, S2). Our study was limited to articles published between 2014 and 2020, and therefore we cannot make inferences about how demographics were cataloged outside of those years. Further, recognizing that university contexts and research experiences vary widely outside of the United States, we intentionally limited this study to articles with data collected from U.S. institutions.
Importantly, because we examined data from published studies, we do not know whether researchers were collecting certain demographic data but chose not to report on it in the publication. We anticipate that researchers would be less likely to collect demographics if their student population had little-to-no expected diversity within the demographic variable (e.g., researchers conducting a study in a class comprised only of traditionally-aged college students are likely to not collect student age). Alternatively, if researchers collected age and did not see interesting patterns, then they may have no chosen to include it. Future studies could explore this by contacting authors of papers and asking them what data they collected and whether they collected data that they chose not to report. The current study attempted a first pass at identifying to what extent demographics are being reported or analyzed in the literature on student research experiences. As such, we took a broad approach of examining many different identities, but did not explore the specifics of how any one identity was collected, reported on, or analyzed. Future research could hone in on specific identities (e.g., gender identity) to characterize how different identities within this category, particularly underserved identities (e.g., nonbinary or women) are represented in the literature on UREs.
While our study examines demographic variable independently, in reality, students in UREs hold many overlapping demographic identities that together impact their experience (intersectionality). Studies on UREs that considered intersectional identities were, for simplicity, coded in our work by the individual demographic identities that each study referenced, and we therefore do not report proportions of studies that considered the intersectional identities of their students. However, a full understanding of how participation in research differentially impacts student will necessitate that future research considers intersectional identities when possible.
Finally, we recognize that access to research experiences, and the demographics of students who participate in research experiences, likely varies significantly across institution types. For example, research-intensive universities typically provide more opportunities for undergraduates to participate in faculty-mentored research experiences than community colleges or primarily undergraduate institutions, and more students from racial and ethnic minorities likely get to participate in research at minority-serving institutions compared with primarily white institutions. We originally intended to investigate how demographics are reported and analyzed by institution type but found these data extremely difficult to consistently code. For example, some studies on the outcomes of UREs specifically revealed the institution where the study was conducted at, some provided an anonymized description of institution type, and others did not explicitly state any information about the institution where their study was conducted. Many studies analyzed data from UREs conducted at multiple institutions, adding another barrier to meaningful interpretation of how institution type impacts reporting and analysis of student demographic data.
CONCLUSION
Educators have increasingly adopted principles of backwards design in reforming STEM classes in higher education—the theory being that we should first identify our desired results (learning outcomes/goals), then identify how we will collect evidence that evaluates whether we achieved our results (assessments), and finally should develop the instructional plans that will align with our preidentified plan for how we will assess whether students have met the desired learning outcomes (Wiggins and McTighe, 2005). However, as a field, STEM educators have not applied the principles of backwards design toward their approach to increasing participation in UREs. If our desired results are that we want students to participate in research because we believe it will support diversity, equity, and inclusion in undergraduate STEM education, we have moved forward with efforts to increase participation of students in research experiences without sufficient effort or intention to collect evidence that evaluates whether we are actually supporting diversity, equity, and inclusion. This study highlights that we know little about the extent to which students with identities beyond race and gender access UREs and how successful they may be in these experiences. Therefore, as we continue to work toward our goal of supporting persistence of all students in STEM, we must strengthen our plans to assess whether and how UREs can benefit students with underserved identities.
ACKNOWLEDGMENTS
We acknowledge Dominic Castelvecchi, Michael Gerbasi, Sophia Hughes, and Julie Roberts for their help with the initial stages of this project. Funding for this project came from a National Science Foundation S-STEM grant (#1644236) that supported our LEAP Scholars. L.E.G. was supported by a