An Exploratory Mixed-Methods Analysis of Factors Contributing to Students’ Perceptions of Inclusion in Introductory STEM Courses
Abstract
In this exploratory mixed-methods analysis of students’ perceptions of inclusion in introductory STEM courses for STEM majors, we asked students to rate inclusion in their class and to provide an open-text explanation of their rating. Analyzing 1930 qualitative responses resulted in a codebook containing academic, identity, and nonspecific categories. The majority of responses (>80%) cited academic factors such as interactions between students and instructors or course elements and policies. Most academic responses aligned with evidence-based teaching practices fostering inclusion, describing a range of strategies and policies instructors can implement to increase students’ perceptions of inclusion. A small number of student responses indicated that their perception of the required knowledge background for the course impacted course inclusivity. Few differences in frequency distributions were found between subgroups examined (gender, race and ethnicity, self-reported inclusion score, and discipline). Additionally, tracking a subset of students (135) across three courses revealed that most (80%) cited different factors influencing their perception of inclusion in each course. This suggests students’ perceptions of inclusive practices are complex, and most students recognize multiple factors that influence their inclusion. Overall, our findings suggest instructors can significantly influence students’ perceptions of inclusion by using multiple inclusive teaching strategies and course policies.
INTRODUCTION
As higher education has embraced the importance of diversity, equity, and inclusion (DEI) in the classroom, education research has begun to identify how to create classroom spaces where everyone has equal access and opportunity to thrive and succeed (Dewsbury and Brame, 2019; Dewsbury, 2020; White et al., 2021). Seven categories of practices that reduce equity gaps have emerged from research on inclusive practices in the classroom (White et al., 2021): employing active learning and group work, validating students’ scientific identities, fostering a sense of belonging, proactively monitoring students’ well-being and performance, cultivating relationships, and promoting resilience and persistence with a growth mindset. This wide variety of research-identified inclusive practices, which include teaching strategies, course design elements, social psychological interventions, and interpersonal interactions, indicates that inclusion is complex and many factors can foster (or inhibit) classroom inclusion. Much evidence supports the idea that using researcher-identified inclusive practices (White et al., 2021) increases students’ satisfaction and success, and results in more equitable outcomes. In general, supportive classroom environments have been shown to improve student belonging, affecting a student's level of engagement, motivation, and, ultimately, achievement in a course (Zumbrunn et al., 2014). For example, studies conducted via survey analyses in engineering courses across multiple universities have shown that inclusive and equitable teaching practices have a greater influence on students’ STEM performance and confidence in their abilities to pursue STEM careers than the students’ own background characteristics (e.g., gender, race and ethnicity, or parental education; Cabrera et al., 2001; Colbeck et al., 2001). Furthermore, whether an instructor endorses a growth (vs. fixed) mindset culture is a stronger predictor of achievement and motivation than any other instructor characteristic (e.g., gender, race/ethnicity, and teaching experience; Canning et al., 2019). Other work has found that students in inclusively taught sections of introductory biology earn higher grades and are more successful in subsequent courses than their peers who had taken the sections without inclusion-based pedagogy (Dewsbury et al., 2022). Some examples of such inclusive teaching practices include adding course content like scientist spotlights (Schinske et al., 2016) and diverse-scientist examples (Handelsman et al., 2022), implementing inclusive instructor language (Tanner, 2013) and verbal/nonverbal instructor immediacy (Liu, 2021), developing a sense of community and making the large classroom seem less intimidating (Tanner, 2013; White et al., 2021), and incorporating teaching practices such as active learning (Eddy and Hogan, 2014) and group work (Clements et al., 2022; Frey and Lewis, 2023). Social-psychological elements (e.g., belonging, growth mindset, and motivation) also influence the performance and retention of students, especially for groups that are marginalized in STEM (Tibbetts et al., 2016; Fink et al., 2018, 2020; Chen et al., 2021; Edwards et al., 2022, 2023). Importantly, research has demonstrated that the incorporation of social-psychological interventions can reduce disparities. For example, using instructional practices aimed at reducing stereotype threat led to more equitable performance outcomes for women in an introductory calculus-based physics course (Kreutzer and Boudreaux, 2012).
While these research-identified inclusive practices have been shown to improve the performance and retention of students in STEM, this research does not address students’ perception of course inclusivity and how these perceptions might vary among students with different identities or lived experiences. Dewsbury and Brame (2019) stress the need for research that captures how students explicitly describe what makes the classroom inclusive for them and how perceptions might vary among students with different identities or academic backgrounds.
To address this gap, the current study focuses on student perceptions of their inclusion in large introductory STEM courses because they are typically the first courses STEM-interested students encounter at the university and often are an important factor in a student's decision to continue pursuing a STEM degree. Student performance and affective outcomes (e.g., belonging, growth mindset, and engagement) in these courses can greatly impact students’ interest and retention in STEM career paths (Good et al., 2012; O'Keefe, 2013; Stout et al., 2013; Sithole et al., 2017; Rainey et al., 2018; Wilton et al., 2019). To achieve the goal of equitable outcomes in such courses, many instructors seek to foster an environment where all students can thrive. Understanding student perceptions of factors that influence their inclusion in the classroom is one important component that can guide instructors in creating learning environments that promote the success and retention of students from all backgrounds in STEM.
In this study, we analyzed data collected from STEM and prehealth students on their perceptions of inclusion in five large introductory STEM courses (introductory biology I, general chemistry I and II, and introductory physics I and II). At the end of the semester, students rated their course inclusivity and explained their scores in open-text responses. Specifically, our study examined their responses through two different lenses. We first examined the overall data set.
Research Question 1: What are the primary factors that students describe when asked about course inclusion? What are the primary factors that are most important for various subgroups (specifically gender, race/ethnicity, and those who report low vs. high course inclusion)?
Second, we explored the data through a second lens by examining a cohort of students who took the three introductory STEM courses (introductory biology I and general chemistry I and II) that are the typical sequence for life-sciences majors in their first year.
Research Question 2: Is a given student's perception of the factors that are important for course inclusivity fixed, or does their perception differ depending on the course? The responses of each student in the cohort were compared across three courses (introductory biology I and general chemistry I and II) for consistency in a student's responses across the three courses.
We recognize that sometimes the interpretation of these types of quantitative analyses can reflect a deficit mindset and can downplay the role of societal inequities, especially when academic performance is considered (Shukla et al., 2022). While we are investigating the frequencies of categories reported by different subgroups based on gender, race and ethnicity, student-reported course-level inclusion score and discipline, our goal is to identify strategies instructors can use to improve inclusion that will help all students thrive in these introductory STEM courses and remain in STEM.
METHODS
Data Collection
The data analyzed for this study were collected over two semesters from five large in-person introductory STEM courses at a selective research university in the Midwest United States. Data were collected in the Fall 2018 semester in General Chemistry 1 and Introductory Physics 1, and in the Spring 2019 semester in Introductory Biology 1, General Chemistry 2, and Introductory Physics 2 (see Figure 1). These were large courses (500+ students/course) with multiple sections taught by different instructors. However, in each course, the instructors worked as a team in which the students in all sections took the same exams, homework assignments, and quizzes (if applicable) and had the same grading scheme. These courses are taken by many different STEM majors and tracks, including prehealth/life sciences majors. The typical sequence for STEM majors is students take General Chemistry I in the fall of their first year and General Chemistry II in the spring of that year, along with Introductory Biology I. For Introductory Physics I or II, their major and/or track dictates when they take the course. Students in the engineering, physics, or architecture tracks take this course sequence in their first year, while students in the chemistry track take this sequence in their second year, and biology/prehealth students take this sequence in their third year. Exact enrollment numbers can be found in Part A of the Supplemental Materials; more details on the courses are in Part B of the Supplemental Materials.
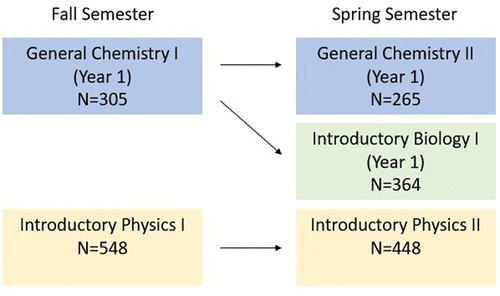
FIGURE 1. The five courses surveyed. N is the number of nonblank, substantive responses per class.
All students enrolled in the participating courses (N = 3051) were invited to participate in this study, approved by the university's Institutional Review Board (IRB #201408004). Near the end of each semester in the 2018–2019 academic year, all students received an email via Qualtrics (Qualtrics, Provo, UT) asking them to complete a packet of surveys, in which one component was an inclusion survey that asked the two questions we analyzed in this study. In return for completing the survey packet, students received some form of extra credit in their lecture or laboratory course as compensation for their time. Approximately 91.9% of students (N = 2804) consented to allow their responses to be used in this research study. Students who did not consent to participate in the research study were still eligible for the extra credit in their courses but were removed from the data set. Students could be enrolled in multiple courses. A total of 1024 individual students provided us with 1930 scoreable qualitative responses.
Demographic data from the registrar (gender, race, and ethnicity) were obtained for those who consented. “Gender” from the registrar data refers to the biological sex of the student that is on their birth certificate and students must select either male or female. This was used as an imperfect variable proxy for gender in our analyses. We recognize that not all students identify with the binary gender they were assigned at birth. Race and ethnicity were organized into three categories: Asian, PEER (Persons Excluded due to Ethnicity or Race; Asai, 2020), and White. PEER consists of the following groups: Black or African American, Latine/a/o, Native American, Native Hawaiian, or Other Pacific Islander. While students could report more than one race or ethnicity, they were placed in one category to simplify the analyses. All students who marked any of the race/ethnicity groups under “PEER” were classified as such (e.g., a student selecting Black and White was categorized as PEER; a student selecting Latine/a/o and Asian was categorized as PEER). Of the remaining students, any student who marked “Asian” was classified as such (i.e., a student selecting Asian and White was categorized as Asian). Any student who marked “White” and didn't mark any other category was classified as White. The demographics of all the responses were 48% men (N = 926), 52% women (N = 1004), 38% Asian (N = 724), 18% PEER (N = 349), and 42% White (N = 808), while 3% (N = 49) did not report their race or ethnicity to the registrar (These totals reflect all the scoreable responses received and not the demographics of the unique number of students. Some students had multiple responses because they were enrolled in multiple courses and answered this question in each course).
During the last 2 weeks of each course of interest, students completed a course-specific end-of-semester survey developed by the Center for Integrated Research on Cognition, Learning and Education (CIRCLE). Each end-of-semester survey included a section on course inclusion. These items comprised a Likert-scale question: “How inclusive do you feel the course [insert course name] was overall?” with a scale of “not at all” (1), “slightly” (2), “somewhat” (3), “moderately” (4), to “highly” (5). After selecting a ranking, students were asked: “Please explain your reasoning for your response to the previous question (two to three sentences).”
Responses to the question above or relevant comments from an open-ended general question (Do you have any additional comments or feedback about the inclusivity of [insert course name]?) at the end of the survey were used to develop the codebook (N = 2931 responses). This study focuses on the analyses of the student responses to the question above in the five introductory courses in which there was a nonblank response (N = 2070 responses). Responses (N = 142) having nonsubstantive text (i.e., “NA”, “None”) in the text box were also removed for a final total of 1930 responses analyzed. Although we did not use any additional data from this survey beyond the questions above, the full survey can be found at the end of the Supplemental Materials.
Data Processing Overview
The qualitative coding in this project was conducted in two phases: Code Development and Refinement of the Codebook. Phase III comprised the quantitative frequency analyses. All three phases are outlined in Figure 2.
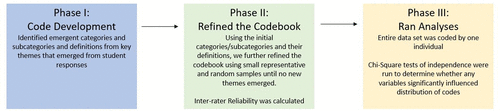
FIGURE 2. Overview of data processing.
Phase I: Code Development.
The team developed a codebook using the inductive qualitative content-analysis method (Hsieh and Shannon, 2005; Elo and Kyngäs, 2008; Cho and Lee, 2014) to identify emergent categories and subcategories. This approach allowed student voices to emerge from the data, highlighting their perceptions of what promoted course inclusion. A.Y. and M.B. independently read through the entire data set of responses generating themes that emerged from the data, and then the research team (A.Y., M.B., and R.F.) met to collaboratively discuss these initial lists of themes and corresponding responses and develop a draft list of combined themes. Each team member brought a different perspective: 1) A.Y. (Ph.D. in biology), a science-education specialist who conducts classroom observations of these introductory STEM courses; 2) M.B., a chemistry undergraduate student who had taken the majority of these courses in prior years; and 3) R.F. (Ph.D. in chemistry), a STEM faculty member who taught the general chemistry courses in other years and a discipline-based education researcher with expertise in qualitative research. This list of combined themes became a rough draft of the original coding scheme with definitions, which was then tested on a randomized sample by M.B., comprising approximately 5% of total responses from each of the six introductory STEM courses. After M.B. independently coded this sample, the research team met, discussed the coding, and adjusted the definitions of both the categories and subcategories based on the coding test run.
Phase II: Testing the Codebook.
To refine this initial set of categories and subcategories with corresponding definitions, A.Y. created additional randomized samples of previously untested responses; one sample with 5% of the total responses and three samples, each with 10% of the total responses, were generated. The five random samples resulted in approximately 40% of the full data set being used in the refining process, which is common practice in qualitative coding (Potter and Levine-Donnerstein, 1999; Krippendorff, 2004). The randomized data sets were iteratively coded by A.Y. and M.B. independently. After each coding session, A.Y., M.B., and R.F. discussed all discrepancies until 100% agreement was reached, and modifications were made to the codebook as needed.
Later in the coding process, the research team expanded to add new members (K.M., H.B., and A.B.). The new members of the team brought different perspectives: 1) A.B., a biology undergraduate student who had taken the majority of these courses in other years; 2) H.B., a physics undergraduate student from a public land-grant research-intensive institution who had taken similar courses at their institution; and 3) K.M. (Ph.D. in biology), a former STEM faculty member and currently engaged in STEM education activities, including serving as a coprincipal investigator on the grant. A.Y. trained the expanded research team by creating two sample data sets with approximately two dozen example quotes from different categories and levels of difficulty. Each member of the new research team individually coded the responses before coming together (A.Y., A.B., H.B., R.F., and K.M.) to collectively agree on one code that best fit each response and make any clarifications to the codebook. Although the coding allowed for a response to be coded into two categories, the vast majority of responses had one central idea. A small sample of responses had two independent ideas, one describing academic and the other identity; hence, these responses were doubly coded for the academic and identity categories.
Once the additional members were trained, A.B., H.B., and A.Y. proceeded to code the five randomized samples containing 5 and 10% of the data to further refine the codebook. After each data set was individually coded, the research team (A.Y., A.B., H.B., R.F., and K.M.) met to discuss all discrepancies until 100% agreement was reached and modifications were made to the codebook as needed. Once these five randomized samples (40% of the data) had been coded with the saturation of the codebook being reached, all five samples were recoded by A.B. and two of these samples were recoded by H.B. using the final version of the codebook. Nominal interrater reliability (IRR) scores for the primary categories were calculated for the academic, identity, and nonspecific categories only due to the rarity of the responses coded as “other”, “do not value inclusion”, and “STEM is objective” (note: the total sum of these three categories was approximately 1.6% of the total data). IRR was calculated also for the academic subcategories only due to the small number of responses that fell into the subcategories of the other primary categories.
For 20% of the randomized data, nominal IRR scores between A.B. and H.B. were calculated using Krippendorf's alpha (Krippendorff, 2004). The three categories tested (academic, identity, and nonspecific) all had values greater than 0.80, indicating a robust level of IRR (Krippendorff, 2004). Robust IRR was not reached across the ‘academic’ subcategories (0.73 ≥ α ≥ 0.48). A table with all the IRR values can be found in the Supplemental Materials (Supplemental Materials Part C). Given the exploratory nature of this study and the robustness of the IRR scores for the primary categories (α ≥ 0.85), the group decided to move on to the next phase of the coding process, which was coding the rest of the data set. These data were individually coded by A.B., and when questions arose, the group (A.B., R.F., and K.M.) met to discuss the questions and reach 100% agreement on the coding.
Phase III. Quantitative Analysis Methods.
Once the data were coded, they were categorized into two types of responses–responses that provided specific information about course elements or responses that were nonspecific in which a general statement was made without any specific factor or practice cited. After this categorization, the data were analyzed using Microsoft Excel and R (R Core Team, 2021). M.C. (Ph.D. in social psychology and data analyst in CIRCLE) ran chi-square tests of independence to determine whether any categorical student variables significantly influenced the distribution of codes (e.g., does the frequency distribution of the Primary Categories differ significantly between men and women?). The three categorical student variables we selected to examine were gender, race/ethnicity, and self-reported inclusion score. For self-reported inclusion scores, we were interested in comparing how students reporting a low or high inclusion score described their experiences. Therefore, we examined these data in a binary manner by separating students into two categories. Those who responded with a three (“Somewhat”) or below on the Overall Inclusion item were categorized as reporting a low inclusion score, and those responding with a four (“Moderately”) or five (“Highly”) were categorized as reporting a high inclusion score. In addition, we examined students’ responses by discipline (i.e., life vs. physical sciences courses), as we hypothesized the gender composition in the classrooms or their intended career path may affect student responses due to negative stereotypes about women in STEM (Wu et al., 2020; Sebastián-Tirado et al., 2023).
Cramer's V was used to determine the effect size of each overall chi-square analysis (0.1-small, 0.3-medium, 0.5-large; Cohen, 1988). We further analyzed significant chi-square tests to locate the sources of the overall effect. First, adjusted residual plots were generated to understand the contribution of each individual cell to the chi-square value. These plots represent, for each cell, how much the actual frequency differed from the frequency that would be expected based on the total number of responses in each subgroup and the total number of responses in each category. From the adjusted residuals, we computed Bonferroni-corrected p values in Excel using the CHISQ.DIST.RT() function to generate the chi-square value and the resulting p value was compared with the new adjusted alpha (our original alpha, 0.05, divided by the number of total comparison tests). The percentage of the chi-square value comes from adjusting for size differences between categories: 100*(residual^2)/(total chi-square statistic).
Because we were concerned that students’ perception of inclusion in a course might be highly correlated with performance, we also examined the relationship between self-reported inclusion groups and performance. We conducted a mixed-effects regression model including data from all courses, with exam performance as the dependent measure, self-reported inclusion group (low vs. high) as a fixed effect, and course as a random effect (i.e., intercepts were allowed to vary across the courses). Exam performance was calculated by averaging midterm and final exam grades for each course. This type of model accounts for the fact that the data have a grouped structure (i.e., students are grouped by course rather than sampled from a single population), with the mean of the dependent measure (exam average) potentially varying across courses. In addition, independent t tests were calculated for each course, with self-reported inclusion group as the independent variable and exam performance as the dependent measure. Cohen's D was used to determine effect sizes (0.2-small, 0.5-medium, 0.8-large; Cohen, 1988).
Cohort for Fixed Versus Situational Perceptions of Inclusion
For the second research question, a unique subset of student responses was used to examine whether a given student's feelings of inclusion were fixed, that is, does a student describe inclusion in the same manner independent of the course? The data set was filtered for students who completed the survey in General Chemistry 1 in the fall semester, and General Chemistry 2 and Introductory Biology 1 in the spring semester. This sample contained 176 first-year students who completed the survey and received a grade in all three courses (N = 528 responses). This unique subset of students provided an opportunity to examine a given student's responses across multiple courses. Further filtering was done based on the responses. Responses from 41 additional students were removed from the analyses because they did not have substantive responses in all three courses, or their responses fell into the nonanalyzed categories (i.e., “do not value inclusion”, “STEM is objective”, and “other”). The final sample size was 135 students (N = 405 responses).
RESULTS
Research Question 1 (RQ1): What are the Primary Factors that Students Cite When Asked About Inclusion in the Course? What are the Primary Factors that are Most Important for Various Subgroups (Specifically Gender, Race And Ethnicity, and those who Report Low Versus High Course Inclusion)?
Students were asked to quantitatively rate the level of their inclusion in the course (1-not at all to 5-highly inclusive) and to give an open-text justification for their score. Quantitatively, students rated their feelings of inclusion fairly highly (i.e., over 75% of students rated their course ‘moderately’ or ‘highly’ inclusive, Table 1), so many of their openended responses were positive in nature.
Class | Average inclusion score (N) | SD |
---|---|---|
Class 1 (Spring 2019) | 3.71, N = 363 | 1.10 |
Class 2 (Fall 2018) | 4.11, N = 305 | 0.94 |
Class 3 (Spring 2019) | 4.10, N = 264 | 0.93 |
Class 4 (Fall 2018) | 4.02, N = 548 | 0.98 |
Class 5 (Spring 2019) | 4.28, N = 448 | 0.83 |
The definitions of the types of responses (i.e., nonspecific and specific), along with example quotes, are found in Table 2; the detailed codebook and example quotes are in the Supplemental Materials (Supplemental Materials Parts E–G). Approximately 20% of the total responses were ‘nonspecific’ in nature (N = 378).
Code | Definition | Quotes |
---|---|---|
Nonspecific (N = 378) | Students described their experience in the course but gave no specific details. |
|
Specific (N = 1552) | Students described their experience in the course with specific details. | See the category and subcategory definitions and example quotes in Tables 3 and 4. |
Impact of Subgroups on Specificity of Responses.
We examined whether the grouping variables in our data (gender, race and ethnicity, and inclusion score group) had any relationships with the two different types of responses (specific and nonspecific). The chi-square test was not significant when examining these two types of responses by race/ethnicity (χ2 = 4.33, df = 2, p value = 0.1145; Cramer's V = 0.048; Supplemental Part H, Supplemental Figure 1) or gender (χ2 = 3.72, df = 1, p value = 0.054; Cramer's V = 0.044; Supplemental Part H, Supplemental Figure 2). We found a significant interaction between the specificity of responses and low (1–3)/high (4 and 5) overall inclusion scores (χ2 = 12.09, df = 1, p value = 0.0005; Cramer's V = 0.079), although the effect size was negligible (i.e., Cramer's V < 0.10; Figure 3). The response specificity distributions for the high and low inclusion groups are categorized into nonspecific responses (High: 21%; Low: 14%) and specific responses (High: 79%; Low: 86%), as shown in Figure 3. After the Bonferroni correction was applied (new threshold p ≤ 0.0125), cell-wise posthoc analyses confirmed that self-reported course-inclusion score significantly influenced the frequency of the nonspecific code (p = 0.006, contributing 63.3% of the chi-square value; Supplemental Part H, Supplemental Figure 3). That is, students reporting low-inclusion scores were more likely to describe specific reasons for their inclusion scores.
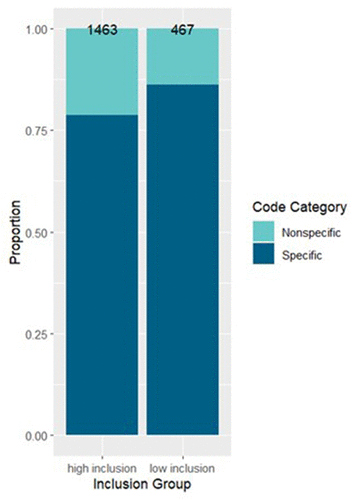
FIGURE 3. Specificity of responses by low/high inclusion group.
Primary Categories.
The specific responses were analyzed to identify the factors students cited as contributing to their inclusion score (N = 1552). Definitions and example quotes from the primary categories (i.e., academic, identity, STEM is objective, do not value inclusion, and others) are found in Table 3. The detailed codebook and example quotes are in the Supplemental Materials (Supplemental Materials Parts E–G). Thirty-one responses fit into three categories that provided no specific information about inclusive practices: ‘other’, ‘STEM is objective’ and ‘do not value’ primary categories (i.e., 1.6% of the data). These responses were removed from further analyses due to the small numbers in each category and their noninformative nature. The remaining specific responses (N = 1521) were analyzed and grouped into three primary categories (academic, identity, and the responses that were doubly coded for academic and identity) and then into subcategories.
Category | Definition | Quotes |
---|---|---|
Academic (N = 1247*) | Student referenced teaching practices and course design elements, and the roles they played in how students engaged in and felt a part of the learning process. This was described by discussing the environment, the opportunities students had to participate, and whether evidence-based teaching practices were used. | “My professor is a great at keeping the class engaged. He is able to get a lot of participation.” “When we would have clicker questions, it would be very inclusive and facilitated a lot of discussion amongst my peers. It would feel like 1 homogenous class, not like we were all separate people just there and listening to one person talk.” |
Identity (N = 243*) | Student refers to a person's identity and the role it played in how involved the students felt in the learning process. This was described through language, course content, or in a capacity related to the individuals involved in the course. | “I was impressed at how Dr [] includes their preferred pronouns in their email signature. It's a small gesture, but can go a long way for some students” “The professors made sure to point out contributions made by women when it pertained to the class.” |
STEM is objective (N = 15) | Students expressed ideas that the STEM material is objective and therefore not influenced by social context and norms. | “There is honestly nothing that is not inclusive about the biology lecture. After all, it is a biology class where most information is factual and objective.” “The discussions were focused on chemicals not people, which makes it hard to be outwardly exclusive.” |
Do not value inclusion (N = 6) | Students do not value the incorporation of inclusion and equity in a STEM course. | “There was a clear effort made to be “inclusive” but it was way too off-putting. I just want to learn about biology, I just want to learn about the information. Inclusivity is not important to me in the context of my bio lecture. I even felt that some of my learning was sacrificed in an effort to express diversity.” “don't care about it” |
Other (N = 10) | The student did not discuss their classroom experience or they discussed a different class. | “Because we only had lab lecture once a week for one hour, I felt that it was not enough time to fully understand what we needed to in class…” “I was very sleepy in the mornings so I wasn't able to completely pay attention.” |
Over 80% of the analyzed responses described ‘academic’ factors when asked to explain their quantitative inclusion score (82%, N = 1247), citing such factors as student-instructor interactions, classroom environment, or teaching practices (Figure 4). One-sixth of the analyzed-responses data set (16%; N = 243) cited ‘identity’ factors, referring to demographic and background characteristics or professor language and course material referring to identities. Approximately 2% of responses were double-coded for the academic and identity categories (N = 31). Overall, for the primary categories, students’ responses about what influenced their perceptions of inclusivity consistently followed a similar pattern across all the courses analyzed.
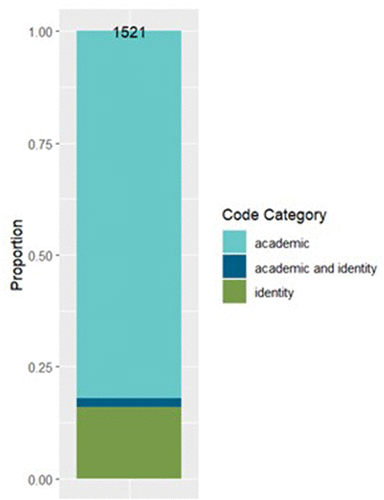
FIGURE 4. Overall breakdown of qualitative data across the primary categories.
We also examined whether the group variables in our data (race and ethnicity, gender, and inclusion score group) had any relationships with the primary categories. We found significance in the gender subgroup (χ2 = 22.2, df = 2, p value = 1.502e-05; Cramer's V = 0.12), although the Cramer's V indicates only a small effect size (0.1 < Cramer's V < 0.3). Race/ethnicity (χ2 = 5.62, df = 4, p value = 0.229; Cramer's V = 0.044; Supplemental Part H, Supplemental Figure 4) and self-reported inclusion score (χ2 = 3.33, df = 2, p value = 0.190; Cramer's V = 0.047; Supplemental Part H, Supplemental Figure 5) were not significant.
Gender in the Primary Categories.
Figure 5 shows the primary-category distributions by gender, separated into academic (Men: 87%; Women: 78%), academic and identity combined (Men: 2%; Women: 2%), and identity (Men: 11%; Women: 20%). Chi-square tests indicate that women were more likely than men to cite ‘identity’ factors in their responses (20% of total responses for women versus 11% for men; Figure 5). After the Bonferroni correction (new threshold p ≤ 0.0083) was applied, cell-wise posthoc analyses confirmed that gender significantly influenced the frequency of using the identity code, with men using the code less frequently than expected (p = 0.002) and women using it more frequently (p = 0.003; Supplemental Part H, Supplemental Figure 6). Within the identity subcategories, one major descriptive difference stood out between men and women. For women, almost 40% of the responses in the identity category (N = 62) described ‘knowledge background’, compared with only 29% (N = 23) of the men's responses. This descriptive thread emerged when students addressed the level of prior knowledge they believed was necessary to be successful in the course or referenced statements instructors made about their own assumptions of the class's prior knowledge.
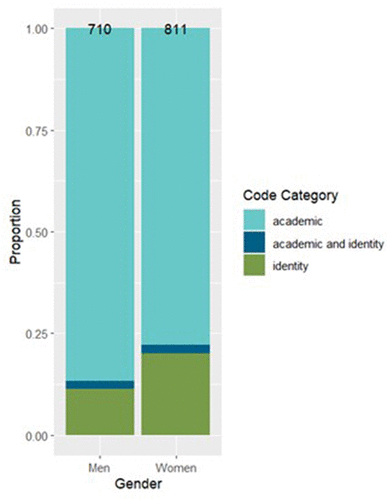
FIGURE 5. Primary categories partitioned by gender.
Despite the significant differences between the distributions of primary categories for gender within the overall data set, both men and women overwhelmingly described academic factors such as student-instructor interactions and course structure when citing reasons for their inclusion scores. Therefore, the academic category was examined in greater detail.
Academic Subcategories.
Subcategories within the ‘academic’ category were defined and examined to further understand the types of teaching and course policy practices that contributed to the students’ perception of inclusion. Three major subcategories emerged from our analyses: ‘environment’, ‘student-instructor interaction’, and ‘course structure’. Additional descriptive threads were examined in both the ‘student-instructor interaction’ and ‘course structure’ subcategories; ‘student-instructor interaction’ responses were examined for how students perceived the interaction (positive, neutral, or negative), while ‘course structure’ responses were examined for whether the factors were more influenced by the instructors or the university/department. Detailed definitions and example quotes for the ‘academic’ subcategories are in Table 4. (Supplemental Materials Part E–G contain example quotes and detailed definitions of the threads).
Academic subcategory | Definition | Quotes |
---|---|---|
Environment (N = 178) | Student noted the classroom environment with respect to how comfortable the class felt, the amount of collaboration with other students during lecture, the amount of respect there was in class, how engaging the lecture was, how supported the students felt, or the overall atmosphere of the classroom. | “The professors made an effort to ensure that both people from the front, sides, and back of the classroom could hear and engage with the material.” “[Instructor] made it very clear on the first day of that class that chem 111 was not a class where we push others down. She said look to your left and right, each person will make it through chem 111. I think this was really powerful.” |
Student-Instructor Interaction (N = 591) | Student addressed the ways in which the instructor(s) encouraged participation, how equal they felt participation was, or how comfortable they personally felt answering/asking questions in the classroom. | “professor encouraged lots of interacting and made sure to mention that anything asked or thought is fine. I felt even if I said the wrong answer no one would judge” “I think the professors made efforts to have everyone feel comfortable with varied examples for everyone. Including having each “third” of the class answer questions so that people would participate more.” |
Course Structure (N = 509) | Student referenced the aspects of the course design or infrastructure that affected the inclusivity. | “I really enjoyed the clicker questions. It gave the students a voice and a way to interact during class.” “[Introductory Biology] offers many opportunities to receive help when you are struggling. The study halls and BTLs provide guidance and extra help to students and allow students to work through problems.” |
Forty-six percent (N = 591) of the responses in the ‘academic’ category described ‘student-instructor interaction’ as a factor in inclusion (denoted interaction in the Figure 6). The vast majority of student-instructor interaction responses (71%, N = 421) described it in a positive way (“The professor would always ask for participation from the audience…”). Approximately 40% (N = 509) of responses were coded as ‘course structure’, and 14% (N = 178) described the ‘environment’ of the classroom. The majority of the responses attributed to the ‘course structure’ subcategory focused on factors within the instructor's influence (81% of course-structure responses, N = 410), such as teaching practices (e.g., incorporating clicker response systems) or course resources (e.g., a peer-led collaborative learning supplemental program, PLTL), rather than factors that were more controlled by the university or department (19% of course-structure responses, N = 99), such as class size. All but one of the ‘university-controlled’ course-structure responses were about the size of the class. Even in the courses with the largest size sections, at most, the class-size responses were 16% of the total ‘academic’ category responses. Students who gave low-inclusion scores were more likely to cite class size than those who scored course inclusion high (15 vs. 6%, respectively, Supplemental Material Part H, Supplemental Figure 10); however, these differences did not achieve statistical significance. Therefore, class size did not emerge as a prominent theme in students’ perceptions of inclusion (or lack thereof). Importantly, greater than 90% of the practices and factors that fell into the academic category were under the instructors’ influence. Only 8% were factors over which instructors had no control.
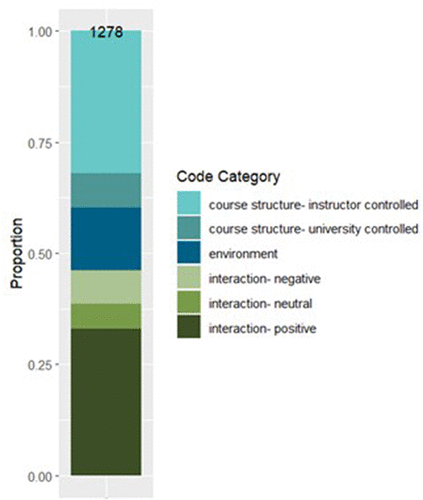
FIGURE 6. Breakdown of the academic category by subcategories.
We also examined whether the group variables in our data (race and ethnicity, gender, and inclusion group) had any relationships with the academic subcategories (environment, student-instructor interaction, and course structure). The results did not significantly differ across gender (χ2 = 4.61, df = 2, p value = 0.10; Supplemental Part H, Supplemental Figure 7). Chi-square tests of independence confirmed that the distribution of academic subcategories differed significantly for the group variables race and ethnicity (χ2 = 12.86, df = 4, p value = 0.01; Cramer's V = 0.072) and low/high inclusion-reporting score (χ2 = 8.86, df = 2, p value = 0.01; Cramer's V = 0.084), with negligible effect sizes. For both subgroups, however, none of the individual cell comparisons reaches significance after applying the Bonferroni corrections (see Supplemental Part H, Supplemental Figures 8–11).
Biology Compared to Other STEM Courses.
We compared the data collected in the introductory biology course to that collected in the physical sciences courses. We hypothesized that biology responses might differ because the composition of the biology course is women-majority, and it contains the largest population of prehealth students and life-sciences majors. Significant differences were found for the primary categories across disciplines (Biology vs. other STEM (chemistry and physics); (χ2 = 8.94, df = 2, p value = 0.011; Cramer's V = 0.077; Supplemental Materials Part H, Supplemental Table 2), but no individual cell frequency differed significantly from the expected frequency after a Bonferroni correction (new threshold p ≤ 0.0083) was applied (Supplemental Materials Part H, Supplemental Figures 12–16 and Supplemental Table 2). We also examined the distribution of responses in the academic subcategories across disciplines (i.e., Biology compared with the physical sciences). The percentages of responses falling into academic subcategories also did not differ across disciplines (p = 0.53; see details in the Supplemental Materials Part H). In addition, for the primary categories within the biology course data, the subgroups (gender, race/ethnicity, and reported inclusions core) were independently examined for differences in the distributions, and no significant differences were found. While the distribution pattern across gender in these data is similar to that seen in the overall data set (in which significant differences were observed), differences across gender were not significant in these biology data, potentially due to the small N and, therefore, a lack of power in our analysis. These data suggest that neither the women-majority composition of the course nor the concentration of prehealth/life-science majors strongly influenced the way students described their inclusion in this large introductory biology course.
Demographics of Students Reporting a Low-Inclusion Score.
To better understand factors that might contribute to variation in students’ perceptions of course inclusion, we asked whether there was any pattern in the demographics of students who rated their courses as low in inclusion (1–3 out of 5). Descriptively, women seemed slightly more likely to rate their courses lower as compared with men, and students who identify as White appeared less likely than their Asian or PEER colleagues to rate their courses lower (Table 5). However, chi-square tests of independence did not show significant differences across inclusion groups for gender. Chi-square tests confirmed that the distribution of high and low inclusion scores differed significantly across race/ethnicity subgroups (χ2 = 10.6, df = 2, p value = 0.004; Cramer's V = 0.08), but cell-wise posthoc analyses showed that race/ethnicity did not significantly influence the frequency of either proportion of low or high inclusion scores after a Bonferroni correction (new threshold p ≤ 0.0083) was applied.
Demographic group | Percentage of students rating inclusion higher (4 to 5 out of 5) | Percentage of students rating inclusion lower (1–3 out of 5) |
---|---|---|
Men | 76.6% | 23.4% |
Women | 75.1% | 24.9% |
–––- | –––- | –––- |
Asian | 74.3% | 25.8% |
White | 78.8% | 21.2% |
PEER* | 70.2% | 29.8% |
We found some interesting trends when examining separate demographic groups that fall within the PEER category. A separate analysis of Latine/a/o students compared with others in the PEER category, using the chi-square test of independence by inclusion-score group, showed that Latine/a/o students rated the inclusivity of their courses similarly to White and Asian students, while the other PEER students (80% Black or African American, 5% multiple races, 15% Native American, Native Hawaiian or Pacific Islander) were more likely to rate their inclusion lower (Part H of Supplemental Materials, Supplemental Figure 17). A chi-squared test showed significance for differences between inclusion scores for race/ethnicity (p = 0.0005), and after a Bonferroni correction, other PEER students were found to be more likely to have a low inclusion score (p = 0.002, contributing 53.1% of the chi-square value; Part H of Supplemental Materials, Supplemental Figure 18). We have not separated Latine/a/o from the PEER subgroup for other analyses (e.g., gender) due to the size and power constraints of our data set; that is, there would be some categories in which the sample size would be too small to make the analyses meaningful.
Effect of Performance on Inclusion Score.
Poor exam performance could affect students’ feelings of belonging to a course, leading to lower inclusion ratings. To determine whether there was any strong relationship between exam performance and overall rating of inclusion, student's overall average exam score was compared between low and high inclusion-reporting groups, using a mixed-effects model with the course as a random variable. There was a significant difference between the low and high inclusion-reporting groups in average-exam performance (F(1) = 36.22, p < 0.001; high inclusion mean = 79.14; low inclusion mean = 76.83), meaning that performance correlated with perceptions of inclusion to some degree. Because a significant effect was found, individual t tests were run for each course to determine whether these results were course dependent. Three out of the five courses examined showed significant differences in average exam performance between the high- and low-inclusion reporting groups (p ≤ 0.03; Supplemental Materials Part H, Supplemental Table 3). Two of those three courses had a small effect size (Cohen's D ≤ 0.32), and one had a medium effect size (Cohen's D = 0.50). Because the proportion of responses that fell into the academic primary category was such a large fraction of the data, we also compared exam scores and inclusion ratings for students whose responses fell into the academic primary category. Similar patterns as with the entire data set emerged, in which the differences in average exam scores were significant between low and high inclusion-reporting groups (F(1) = 23.94, p < 0.001; high inclusion average: 80.40; low inclusion average: 76.39). Therefore, average exam performance was correlated with a student's feelings of their inclusion in the course, and the same three courses that drove the significant differences in the whole data set also were significant in the academic subcategories data set (p ≤ 0.05; Supplemental Part H, Supplemental Table 4). Despite observing some correlation, given that the correlation was not universal across all the courses examined and the effect sizes were small or medium, this correlation is weak. The lack of a strong correlation suggests that performance is not a dominant factor in student perceptions of inclusion. Rather, students found other factors to be influential.
Research Question 2 (RQ2): Does A Given Student Cite the Same Primary Categories and Academic Subcategories Across The Three Courses or Does What a Student Perceives as Important for Inclusion Differ Across the Three Courses?
In the second research question, we tracked a cohort of the same first-year students in their first two semesters in a life-sciences track across three introductory STEM courses (General Chemistry 1 in the fall semester, and General Chemistry 2 and Introductory Biology 1 in the spring semester) to examine how these students perceived their course inclusion. There were 135 students who completed all three courses and completed the survey for each course with substantive responses (N = 405 responses).
Cohort Data.
Comparing this cohort sample to the entire data set, the demographics differed, but the average reported inclusion scores were similar (Course 1: 4.12 overall vs. 4.16 subset; Course 2: 4.08 overall vs. 4.11 subset; Course 3: 3.70 overall vs. 3.80 subset). Demographically, this subset of students had an overrepresentation of women (60% vs. 52% women in the overall data set) and students who identified as Asian (47% vs. 39% overall data set). Men (40% vs. 48% in the overall data set) and students who identified as White (35% vs. 43% in the overall data set) or PEER (15% vs. 19% in the overall data set) were underrepresented in this subset. In both data sets, 3% of students did not report their race/ethnicity.
In RQ2, the overall pattern of responses falling into major categories echoed those seen in RQ1 (Table 6, below). Slight differences in proportions were found between the primary categories when comparing the whole data set to the cohort subset (p = 0.02; Supplemental Materials Part I, Supplemental Figure 19), but no comparison was significant after a Bonferroni correction. No significant differences were found among academic subcategories proportions (p = 0.61; Supplemental Materials Part I, Supplemental Figure 20) or between the non- versus specific response proportions (p = 0.90). There were no significant differences between the different groups (race/ethnicity, gender, overall low/high reported inclusion scores) in Research Question 2 (Supplemental Materials Part I, Supplemental Figures 21–23). The lack of significant differences may be due to the smaller sample size of RQ2 (RQ2, N = 405; both the analyzed specific and nonspecific responses in RQ1, N = 1899). In general, the patterns of first-year students’ responses were similar to the patterns for the total population. That is, academic factors were very important to students’ perceptions of inclusion in the classroom, regardless of discipline or year.
Category | Proportion in RQ1 | Proportion in RQ2 |
---|---|---|
Nonspecific | 0.20 | 0.19 |
Specific | 0.80 | 0.81 |
Primary Categories (Specific Responses only) | ||
Academic | 0.82 | 0.87 |
Academic and Identity | 0.02 | 0.02 |
Identity | 0.16 | 0.11 |
Comparison of Factors Across the Three Courses for a Given Student.
We examined whether a given student cited the same overall category across these three courses (Supplemental Materials Part I outlines our findings). Twenty percent (N = 28) of these students perceived similar factors (i.e., either the same primary category and subcategory or consistently nonspecific) as important for inclusivity across all three courses. Of the 135 students, only 5% (N = 7) were consistently nonspecific, two (1.5%) consistently cited identity, and 14% (N = 19) consistently cited the same academic subcategory. However, a majority (80%, N = 107) described their perceptions of inclusion differently across the three courses. Of the 107 students who cited different categories of factors across the three courses, 44% (N = 47) sometimes were specific and sometimes were nonspecific, 39% (N = 42) cited factors that fell into different academic subcategories, and 17% (N = 18) sometimes cited academic factors and sometimes cited identify factors.
Sixty percent of students (N = 81) were always specific in their responses; Of those 81 students, 78% (N = 63) described the same primary category in their responses for all three courses, with the vast majority in the academic category (N = 61) with only 2 in the identity category. However, of these 61 students, only 31% (N = 19) cited factors that fell into the same subcategory for all three courses. Almost two-thirds (63%) of these repeated responses fell into the student-instructor interaction subcategory (N = 12), followed by the course structure (N = 6), and environment (N = 1) categories. Most importantly, the vast majority (69%, N = 42) of the students consistently identifying academic factors across all three courses cited factors that fell into different academic subcategories. The dominance of students citing academic factors in this analysis and the prominence of student-instructor interactions being important for students’ perceptions of inclusion is consistent with findings in RQ1, where academic-related factors and student-instructor interactions strongly influenced students’ perceptions of inclusion. Overall, because the vast majority of the students, 80%, described their perceptions of inclusion differently across the three courses, our results support the idea that most students do not have a fixed perception of what makes a course inclusive and that students’ perceptions, of course, inclusivity are complex
DISCUSSION AND IMPLICATIONS
Student Perceptions of Their Inclusion in Introductory STEM Courses
In this exploratory mixed-methods study, we asked students to rate their perceptions of inclusion in their large introductory STEM courses (general chemistry I and II, introductory biology I, and introductory physics I and II) and to explain their reasoning for their rating. Most students (∼75%) found these courses moderately or highly inclusive. While twenty percent of the total responses were ‘nonspecific’ in nature (i.e., “It never felt uninclusive”), eighty percent of the total responses were specific and many of the specific factors that students cited align well with published literature on inclusive and equitable practices (Dewsbury and Brame, 2019; White et al., 2021).
Students described their perceptions of inclusive practices or factors in multiple ways, recognizing many elements that researchers have shown to affect performance and retention (for a review, see White et al., 2021). Most of the student responses (80%; Figure 4) fell into the academic primary category; these comments referred to group activities, active learning, course policies, and social psychological concepts such as belonging and a growth mindset. To our surprise, only 20% of the responses referred to identity. This high percentage of responses referring to academic factors and a much smaller percentage of responses referring to identity was also observed in two other studies exploring student responses on course inclusivity in large general chemistry and introductory physics courses at a large research-intensive public institution (Walker et al., 2024; Bustamante et al., 2024). Our expectation was that identity would be a more prominent category because of the extensive literature on the positive impact of using diverse examples (Handelsman et al., 2022) and scientist spotlights (Schinske et al., 2016). While students highlighted many different course-specific elements in their responses, the proportions of responses that fell into the academic and identity categories and academic subcategories across the different courses were very similar (Supplemental Materials Part H, Supplemental Table 2). Regardless of course/discipline, students were consistently more likely to cite academic factors than any other primary category, and the proportions of responses in each primary category across courses are similar. In addition, few differences in the proportion of responses in the categories were seen across the subgroups we analyzed (i.e., race and ethnicity, gender, and course-level inclusion score). We only saw significant differences in the distribution for gender, where the proportion of ‘identity’ category responses was higher in women and lower in men, and for course-level inclusion score, where those students who rated course inclusion low gave less ‘nonspecific’ responses. This higher proportion of women discussing identity in their responses is similar across all the courses, regardless of the gender composition. Possibly, this consistent difference between men and women might be due to negative stereotypes about women in STEM (Wu et al., 2020; Sebastián-Tirado et al., 2023). The ‘academic’ category is overwhelmingly the most prominent primary category across all subgroups, suggesting that all students can benefit from instructors’ efforts to use inclusive strategies.
Academic Factors Focused on Practices Within the Instructor's Influence
Of the responses in the ‘academic’ primary category, greater than 90% cited factors within an instructor's influence. Almost half of the responses in the academic category were centered around student-instructor interactions, such as encouragement of participation in the classroom, the amount of equity in participation, or how comfortable students felt participating (Figure 6). Students clearly value instructors who create an environment that facilitates active participation and these practices are important to student perceptions of an inclusive classroom. The large number of responses that cite participation as important for an inclusive classroom environment resonates with research that indicates verbal participation becomes more difficult or decreases for some groups of students as class size increases (Eddy and Hogan, 2014; Eddy et al., 2015; Nadile et al., 2021; York et al., 2021). Our data amplifies and extends these previous studies. The positive nature of many of these comments indicates that instructors can be effective in fostering an inclusive environment by encouraging participation, even in large introductory STEM courses, where some students may feel intimidated.
The majority of the remaining ‘academic’ responses described instructor-influenced aspects of the course structure, such as course resources or pedagogical strategies. This includes using clickers and small-group work and regulating the pace of the class or classroom environment (e.g., how engaging the lecture was, the amount of collaboration with other students during the lecture and the classroom atmosphere; Figure 6). These are practices and strategies that are documented in the literature as helping to create an inclusive and equitable environment (White et al., 2021). Only a few students (Figure 6) described factors outside of the instructor's influence, with all but one of these responses focused on class size. Research suggests that large class sizes can negatively affect learning and engagement (Cuseo, 2007). However, despite the fact that the average course size we surveyed was fairly large (Biology: N = 312; Chemistry: N = 231; Physics: N = 98), most students did not perceive class size as a prominent aspect of inclusion, including those who gave low-inclusion scores. Hence, in these courses the vast majority of factors students perceive as influencing their level of inclusion are the teaching strategies, course policies, course resources, and instructor actions. These elements, chosen and implemented by instructors, reflect many practices that research has demonstrated to improve performance and retention. This study, along with previous research, supports the idea that there are many ways instructors can foster inclusion by using teaching strategies that help develop a sense of community in the classroom, promoting participation, and making the large classroom seem less intimidating (Tanner, 2013; White et al., 2021). Specific practices described by students in this study and evidence-based practices supported by the literature include active learning techniques (Freeman et al., 2014), such as small-group interactions and student collaboration (Frey et al., 2018; Clements et al., 2022), implementing instructor immediacy such as faculty developing personal rapport with students through ‘getting to know you’ surveys and informal social interaction before and after class (Liu, 2021), and encouraging students to use office hours by renaming them ‘student hours’ and prompting them to ask questions that go beyond course-specific material (e.g., study strategies and career advice; Smith et al., 2017).
Identity Responses are Less Frequent and Tend to Focus on ‘Knowledge Background’.
It is notable that within the ‘identity’ category, in which students cite ideas related to their or others’ demographics or academic background, a large proportion of responses relate to ‘knowledge background.’ In these responses, students describe their perceptions of an instructor's expectation of their level of previous knowledge of the subject. Responses that describe knowledge background are far more numerous than responses that describe nonperformance-related identities such as gender or race/ethnicity. Prior qualitative work at this institution also identified ‘prior knowledge’ as a factor when students explain their feelings of belonging in General Chemistry (Fink et al., 2023). In other studies exploring student responses about course inclusivity in a general chemistry course and a calculus-based introductory physics course at a large research-intensive public institution, a small percentage of the total responses were coded as identity (Bustamante et al., 2024; Walker et al., 2024), similar to the current study. Looking at the descriptive threads of these responses in a general chemistry course (Walker et al., 2024), which consists of all science majors, engineers, and prehealth majors, the majority of the ‘identity” responses described “knowledge background’, similar to the current study. However, while ‘prior knowledge’ responses are represented in student responses from other courses, the percentage may differ among courses; for example, in the calculus-based introductory physics course (Bustamante et al., 2024), which consists of physical sciences majors and engineers, the ‘identity’ responses were equally distributed among demographics, previous knowledge, and professor language (especially concerning the use of pronouns). Instructor's use of language about perceived prior knowledge has been shown in the literature to factor into inclusivity in the learning environment (Cwik and Singh, 2022). Hence, addressing student concerns about academic preparation early in the course is an important strategy for instructors to consider. This might be accomplished by promoting a growth-mindset environment (Fink et al., 2018; Canning et al., 2019; Muenks et al., 2020). Other strategies include the instructor avoiding communicating to the class their own assumptions about student prior knowledge (e.g., by not skipping steps when demonstrating how to solve problems or not stating that students should already know a specific concept from AP courses) and reassuring students that a diversity of experiences can enrich course discussion and contribute to learning (Svinicki and McKeachie, 2011; see below for further discussion).
Of the subgroups we examined, we found significant differences for gender, where the proportion of ‘identity’ responses was higher in responses from women and lower in responses from men (Figure 5). One might have expected this result because women are typically marginalized in STEM fields. However, of the ‘identity’ responses from women, over 40% described ‘knowledge background’ compared with less than 30% of the ‘identity’ responses from men. This pattern occurred even though many of the courses in this study enrolled 50% or more women, and five of the twelve faculty teaching these introductory courses were women. One hypothesis to explain this difference is that women are more self-conscious about their academic preparation than men, perhaps due to negative stereotypes around women in STEM (Good et al., 2012; Stout et al., 2013) or the higher rates of imposter syndrome seen in women (Vaughn et al., 2020). Previous work has shown that women are less likely than their men colleagues to report feeling comfortable asking questions in large enrollment STEM courses and are more likely to select factors such as anxiety or not feeling that they know the material well enough as the reason(s) that discourage them from doing so (Nadile et al., 2021).
Additionally, identity was more likely to be coded in responses from students who rated inclusion low, with ‘identity’ responses being more numerous than nonspecific ones. Furthermore, of those identity responses, approximately 60% described ‘knowledge background’, similar to the trend seen with responses from women. Therefore, in designing strategies to increase inclusion, it is important for instructors to consider how knowledge background expectations or perceived expectations might differentially affect women and students who feel less included (Cwik and Singh, 2022). Instructors can avoid communicating assumptions about prior knowledge to their students in a variety of ways. For example, instructors can: 1) avoid saying certain material is not covered because it is “review”, 2) ask for multiple voices to provide answers to questions posed to allow for a variety of opinions or problem-solving strategies, 3) promote a safe environment where students can make mistakes through fostering a growth-mindset culture, or 4) communicate their confidence in the student's ability to reach high standards (Tanner, 2013; Theobald et al., 2020; White et al., 2021). Fostering a growth mindset is an important strategy, because an instructor endorsing a growth mindset is a stronger predictor of achievement and motivation than any other characteristic of the instructor (e.g., gender, race/ethnicity, teaching experience, etc.; Canning et al., 2019). Behaviors that were identified as communicating a growth-mindset are offering opportunities for feedback, responding to students who struggle by giving additional support and attention, supporting the idea everyone can learn, and placing value on the learning process (not only outcomes; Kroeper et al., 2022). Instructors can use assignments (e.g., homework) that are graded on effort or completion rather than performance or use exam-wrapper exercises (White et al., 2021). Another strategy that may improve what could be a lack of student self-efficacy is for instructors to use ‘scientist spotlights’, where instructors highlight different pathways people use to become scientists or apply science to their lives (White et al., 2021; Metzger et al., 2023). This can demonstrate to students that becoming a scientist is not a linear path that is only achieved by individuals with specific identities or abilities.
Nonspecific Responses.
We note that the ‘nonspecific’ category was approximately a fifth of our total data set (Figure 3). These nonspecific responses differ from responses in which students left the question blank or wrote nonsubstantive text (i.e., “NA”, “None”). This large percentage of nonspecific responses was also seen in another study exploring student responses on course inclusivity in a large general chemistry course at a large research-intensive public institution (Walker et al., 2024). Why some students did not cite specific factors is unclear. One hypothesis is that if a student feels comfortable and included, they may not actively recognize what contributes to this perception. Students who feel less included may be more likely to examine why they feel that way. Consistent with this idea, students who reported a low-inclusion score were less likely to give responses that were categorized as ‘nonspecific’ than their peers who gave high-inclusion scores (Figure 3). Another hypothesis is that students who gave nonspecific responses have an idea of what made the course feel inclusive but did not take the time to further elaborate on this idea. This survey was administered near the end of a larger suite of surveys, and students might have experienced survey fatigue when completing the survey package. Using a structured set of questions that asks about inclusive practices instead of a single question with an open-ended response would potentially provide more comprehensive information about the impact of inclusive practices.
Perception of Inclusion Is Complex.
RQ2 examined whether a given student described the feelings of inclusion in similar or different manners across multiple courses (i.e., general chemistry I and II, and introductory biology I). Only 20% of students described factors that fell into the same primary and subcategory when discussing inclusivity across the three different classes. These data suggest that while student populations in STEM introductory courses consistently describe inclusion in similar overarching themes, individual students usually cite different factors that contribute to their perceptions of inclusion in each course. A caveat in the interpretation of this finding is that we have only one data point for each student in each course. A student might cite different factors if asked to reflect on inclusion multiple times during a single course, so the differences we identified may not reflect course-to-course variations in student perceptions. While our data do not address this issue directly, one aspect of the data may bear on this caveat: in each course, some students cited factors that were implemented only in that course, such as ‘scientist spotlights’ that were implemented in general chemistry II or lecture demonstrations that were implemented in introductory physics. This suggests that students respond to the different learning environments they experience in each course.
Despite this limitation, overall, the wide variety of different factors students cite across their courses and the tendency of individual students not to cite the same factors in different courses supports the idea that students’ perception of what makes them feel included in a course is complex and influenced by different teaching strategies. Students pay attention to details of course structure, instructor actions, and teaching-strategy choices in each course and multiple different aspects influence students’ perspectives of inclusion. This aligns well with the extensive literature on research-supported inclusive teaching practices (e.g., Theobald et al., 2020; White et al., 2021). Overall, our data should encourage instructors to incorporate multiple research-supported inclusive practices into their courses, as detailed in the RQ1 discussion, and be confident that students notice and appreciate such efforts.
Educating Students on Recognizing Inequity in STEM.
A small number of responses (1.6%) were excluded from this analysis (i.e., the “do not value inclusion” and “STEM is objective” categories) but are worth noting. These responses differ from those that were analyzed in the primary categories of academics and identity because they do not provide specific information on practices that impact perceptions of inclusion. As seen in Table 3, the ‘STEM is objective’ category reflects a student's lack of appreciation that STEM can be biased, while the ‘do not value’ category reflects an attitude that a focus on inclusion in STEM classrooms is not important or necessary. The small number of responses in these categories indicates that such beliefs and attitudes are not common among students. However, they suggest that there is an ongoing need to educate students about the way bias and structural inequities can affect STEM education and STEM fields (Doucette et al., 2022).
LIMITATIONS
A limitation of our work may be that due to the size of our racial/ethnic demographic groups, we do not have the power to detect differences for a single racial/ethnic group. Because of the small number of PEER students in our sample, all PEER students were grouped together for our statistical analyses. The demographic variables were highly simplified for the quantitative component; therefore, the nuances and complexities of gender identity, racial/ethnicity identity, race-gender intersections, and other identities and identity intersections were not captured in the results. In addition, although we studied the variation of the number of responses in each category or subcategory via group, we did not look qualitatively at possible variations in specific practices and strategies students identified within each theme based on the group. These both are important areas for future study.
Because our study focused on large introductory STEM courses, it is important to note that these results may not reflect students’ perceptions of nonintroductory or small introductory STEM courses or non-STEM courses. The size and level of a course often influence the teaching practices used and, therefore, might result in differences in students’ perceptions of inclusive elements.
These data were gathered at a highly selective institution which may influence the patterns we see. However, similar results were obtained in two large introductory courses (chemistry and physics) at a large research-intensive public institution (Bustamante et al., 2024; Walker et al., 2024), suggesting that the categories and themes identified here might be widely applicable.
CONCLUSIONS
The goal of this work was to better understand how students perceived inclusion in their large introductory STEM courses. Understanding the specific practices and course elements students cite as influencing inclusion assists instructors in identifying and implementing strategies to create an inclusive environment in the classroom for all students. We found that the majority of students cite academic factors as important for inclusion, such as interactions between students and their instructors or various course-structure elements. These responses focused mostly on the actions of their instructors and indicated that students value the implementation of evidence-based inclusive practices by their instructors. The responses align well with the literature that has identified inclusive practices and show such efforts make a difference in the student experience in the classroom (Tanner, 2013; White et al., 2021). One theme emerged that has not been prominent in the research literature: Instructor assumptions about students’ knowledge background influenced some students’ perceptions of inclusion. However, students cited a wide variety of different course design components, teaching strategies, and instructors’ behaviors and actions as influencing their perceptions of inclusion, many of which are easy to implement. Few differences in the proportions of responses in the categories/subcategories were observed among the various subgroups examined (gender, race and ethnicity, low/high course-level inclusion, discipline), and those few differences had small effect sizes. This study supports the idea that instructors have many choices as they seek to foster an inclusive classroom environment. These findings should encourage faculty to implement inclusive and evidence-based practices in their teaching, as these efforts are noticed and highly valued by their students.
ACKNOWLEDGMENTS
This research was funded by an Inclusive Excellence grant to Washington University from the Howard Hughes Medical Institute (Award No. 42008723; PI: initially Regina Frey, currently Kathryn Miller). The authors would like to thank the instructors and students in the introductory STEM courses who participated in this study.