Mind the Gap: Investigating Disaggregated Student Performance in an Upper-Division Biochemistry Course Following Team-Based Learning
Abstract
Team-based learning (TBL) is a highly intense active learning pedagogy that uses a cycle of preclass preparation, formative assessment for readiness, mini-lectures, and complex team exercises. Consistent with the literature on active learning, prior research on TBL consistently shows its benefits for student outcomes as compared with previous lecture. However, little work has examined student outcomes disaggregated by demographic variable. We analyzed assessment and student demographic data (i.e., binary gender, racial/ethnic group, generational status) in three semesters of TBL in an upper-division biochemistry course to understand the degree to which performance could be predicted by student demographics. We use theoretical and empirical research from social psychology to hypothesize that the intense interpersonal interactions of TBL could activate psychological threats, the effects of which would be measurable as differences in student performance that correlate with demographic variables. Our regression analysis did not support this hypothesis. This null result invites a deeper discussion on how we measure the potential effects of active learning on student outcomes, particularly given how important it is to account for intersectional and invisible identities.
INTRODUCTION
A hallmark of active learning is student interaction (Driessen et al., 2020). In many active learning classrooms, students are invited to interact with nearby neighbors in transient pairs or small groups (e.g., think-pair-share, peer instruction) which are likely to differ in composition and intensity of interaction from day to day. Team-based learning (TBL) is one type of active learning pedagogy that is distinct in the respect that students are assigned to permanent groups that work together daily. The efficacy of TBL has been demonstrated in a variety of undergraduate contexts, including in majors and nonmajors courses, multiple Science, Technology, Engineering, and Math (STEM) disciplines, and in both introductory and upper-division courses (e.g., St. Clair and Chihara, 2012; Lamm et al., 2014; Hettler, 2015; Yuretich and Kanner, 2015; Behling et al., 2017; Donovan et al., 2018; Parappilly et al., 2019; Lekhi and Varao-Sousa, 2024). However, most studies have generally looked only at course-level aggregate effects. Few have examined outcomes at the student level, limiting our understanding of who benefits from TBL in these contexts.
In TBL, student groups are intentionally formed by leveraging student strengths, backgrounds, and experiences to construct groups with diverse composition (Michaelsen and Sweet, 2008). Diverse group composition is rationalized from a resource perspective; the greater the range of individual perspectives, the greater the quantity of resources available to the group to solve meaningful problems (Watson et al., 1993; Sweet and Michaelsen, 2007). Yet assignment with an eye toward maximum diversity overlooks the potential implications of intense social interactions between students of diverse social identities on group dynamics and performance. More recent research on active learning suggests that students from historically marginalized racial and ethnic groups experience the group work of active learning classrooms as unwelcoming (Nardo et al., 2022).
Reinholz and colleagues (2022) observed gendered performance differences between students of binary gender identities in active learning environments, which their evidence suggested was attributable to gendered differences in engagement. Other studies have noted differences in in-class and group work participation and subsequent performance correlating with historically marginalized identification (Eddy et al., 2014; Eddy and Hogan, 2014). Social psychologists postulate that these differences in performance maybe be explained, at least in part, to interactions with social identity. Several studies have demonstrated that the intersection between a student’s social identity and their social context can produce psychological barriers (e.g., self-doubt, fear of failure) which in some cases can reduce sense of belonging, motivation, engagement, that can affect subsequent academic performance (Walton and Cohen, 2011; Cohen and Sherman, 2014; Easterbrook and Hadden, 2021; Nardo et al., 2022). The implications of these prior studies prompted us to hypothesize that membership in a diverse TBL group might increase the likelihood that students with historically marginalized gender, racial/ethnic, or generational identities would perceive psychological barriers, the effects of which would be measurable as reduced academic performance. Therefore, we sought to answer the following research question:
To what extent are differences in performance in a TBL course explained by students’ historically marginalized identities?
WHAT IS TEAM-BASED LEARNING AND FOR WHOM IS IT EFFECTIVE?
TBL is a high-intensity active learning approach that involves small teams of 5–7 students spending most of class time working collaboratively on complex disciplinary problems. Intense teamwork of this nature requires a certain level of team readiness which is achieved through preclass assignments intended to prepare students for in-class teamwork, individual and team readiness assurance tests (iRATs and tRATs) that diagnose student readiness for teamwork, and brief lectures (as needed) to fill in knowledge gaps (Michaelsen and Sweet, 2008). As the name implies, student-student interaction is the cornerstone of TBL course design. Permanent student teams work on two types of activities, the tRAT and a team activity. Both activities involve discussing and negotiating a consensus response to questions but differ in that the readiness assurance process (RAP) tends to focus on comprehension or simple application problems whereas the team activities require higher order cognitive skills.
TBL has been shown to improve student performance, confidence, motivation, attitudes, teamwork skills, and engagement as compared with other instructional methods.(e.g., Parmelee et al., 2009; Persky and Pollack, 2011; Huitt et al., 2015; Nyindo et al., 2014; Yuretich and Kanner, 2015; Behling et al., 2017; Cho and Kweon, 2017; Jeno et al., 2017; Remington et al., 2017; Parappilly et al., 2019; Sakamoto et al., 2020). However, most of the studies are limited to business, nursing, medical, pharmacy, or other graduate/professional school contexts which are distinct from undergraduate STEM (Haidet et al., 2014). While some studies of TBL report the demographics of their sample (e.g. Parrish et al., 2021), most studies fail to examine disaggregated student data. The disaggregation of student data is an important practice in evaluating educational interventions, including active learning (Bensimon, 2005; Eddy and Hogan, 2014; Theobald et al., 2020). For active learning in general, higher-intensity practices reduce, but do not seem to fully eliminate, gaps in performance between students from advantaged and marginalized backgrounds (Theobald et al., 2020).
SOCIAL IDENTITY THREAT AND STUDENT PERFORMANCE
Social identity can be defined as the thoughts, feelings, and beliefs an individual has about themselves based on their membership in a group (Turner et al., 1979; Hogg and Turner, 1987). In educational contexts, social identity shapes how daily experiences in the classroom are perceived. For example, imagine a female-identifying student in a calculus course that also happens to have mostly male-identifying students enrolled. A poor score on a quiz might be experienced by this student as social identity threat, manifesting in thoughts such as, “Students like me don’t belong here” because of gendered societal messages about math ability and the lack of representation of other female-identifying students. Repeated threats to social identity are theorized to lead to a series of negative recursive events (e.g., reduced motivation, withdrawal) that over time may have negative educational outcomes (e.g., reduced performance, leaving the major; Cohen and Garcia, 2008; Murphy and Zirkel, 2015; Easterbrook and Hadden, 2021). While this example is oversimplified—gender is not binary, identities are intersectional, and negative experiences do not always lead to negative educational outcomes like reduced performance—it does reveal that the ways in which daily experiences are perceived are highly context specific.
The designers of TBL prescribed forming diverse teams based on the principle of resource wealth distribution—the assertion that diverse team composition maximizes the resources available to the group for problem solving (Michaelsen and Sweet, 2008). This approach may also presume a greater likelihood of divergent viewpoints and therefore more careful consideration and deliberation during group tasks. But when viewed through a social identity lens, it is possible the opposite will happen. Some students, particularly those from socially marginalized groups, may experience threats to their social identity that over a semester of intense group work during TBL, produces long-term performance inequalities (Eddy et al., 2015; Hood et al., 2020; Easterbrook and Hadden, 2021).
MATERIALS AND METHODS
We sought to understand the degree to which performance in a TBL course could be explained statistically by students’ historically marginalized identity. To this end, we gathered a variety of student performance measures (i.e., exam scores, course grades) and tested whether performance could be explained by demographic variables.
Study Context
Data were gathered in the first semester of an upper-division biochemistry two-course series. Typical course enrollment ranges between 45 and 65 students, about two-thirds of whom are junior and senior undergraduates, and one-third of whom are graduate students. Graduate students are generally first-year graduate students studying disciplines outside biochemistry (e.g., animal science, chemical engineering) who are required to take the course to improve their biochemistry background knowledge.
In the TBL classroom, students were assigned to groups of four to six. In Fall 2019, groups were composed of both undergraduate and graduate students. In the following two semesters, undergraduate and graduate student were separated into their own groups. Students’ prior academic performance in biochemistry prerequisites, major/graduate discipline, gender, and race/ethnicity were factors used to create diverse groups (Watson et al., 1993), with special attention to ensuring that students with historically marginalized identities were not the only individual of that identity in their group. In Fall 2019 and 2020, the course had the same two instructors. In Fall 2021, one of the instructors was replaced with a new instructor. Additionally, the students worked synchronously online during the Fall 2020 semester in their TBL groups because of the COVID-19 global pandemic (Arneson et al., 2022).
The course structure (Figure 1) was informed by recommendations in the TBL literature (Michaelsen and Sweet, 2008). On the first day of the course, students were introduced to their permanent groups and provided with the rationale for forming permanent groups; students develop as teammates over the semester by completing in-class activities, participating in constructive peer review, and build working relationships to succeed in learning biochemistry (Walker et al., 2020). Students also engaged in a team-building activity focused on behaviors—positive and negative—that are common during TBL group activities. Students identified their own constructive and destructive group behaviors and shared them with their groups with the help of a handout (adapted from Brunt, 1993). As part of these introductory activities, they created paper placards with their name, pronouns, constructive and destructive behaviors they identified, and a personal interest or hobby. Students used these placards to introduce themselves to their TBL team and the instructors, who referred to the placards as a name-, pronoun-, and behavior-learning tool at the beginning of the semester. As a team and with the whole class, students created and shared plans to leverage the team’s constructive behaviors and minimize destructive behaviors.
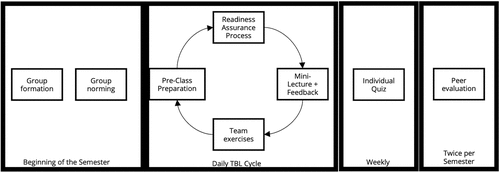
FIGURE 1. The format of Team-Based Learning in the Biochemistry course.
After the first day, the class consisted of 50-minute periods that met 3 days per week (M, W, F); team activities took place each day. iRATs and tRATs were only completed on Mondays and Fridays. Before each day of class, students reviewed instructor-provided materials to prepare for the RAP and team exercises. These materials typically included a short, assigned reading (Nelson and Cox, 2017), slides, or videos. Before class, students started the RAP by completing the iRAT. The iRAT was administered online via the course learning management site (LMS) and was comprised of questions that predominantly targeted knowledge, comprehension, and low-level application cognitive skills (Anderson and Krathwohl, 2001). When they arrived in class, students completed a tRAT in groups on the LMS. In 2019 and 2020, the tRAT was identical to the iRAT. In 2021, a minimum of 50% of tRAT questions were isomorphic to the iRAT questions. After the tRAT, the instructors reviewed student responses to the tRAT to determine any residual gaps in baseline knowledge and, when necessary, provided a brief lecture (∼15 min) to prepare the students for the team activities. TBL groups completed team exercises in class which commonly consisted of more complex activities such as interpreting data, developing hypotheses or explanations for an example phenomenon, or completing a case study through the LMS. As an addition to the conventional TBL format, once per week, students took an individual quiz. All TBL activities contributed to a students’ grade in the course as prescribed to invite both individual and team accountability throughout the course (Michaelsen and Sweet, 2008). The course grading scheme can be found in the Supplemental Materials.
Twice during the course, students completed anonymous peer evaluations for their TBL group members. The peer evaluation included two questions asked about each teammate, “Provide a numerical rating for Student X on a scale from 1 to 10 (with 10 being the most favorable). In your score, consider attendance, preclass preparation and participation in team activities.” as well as “Provide constructive feedback for Student X as a teammate. Consider strengths and provide suggestions for areas of improvement.” The instructors checked peer evaluations to monitor group dynamics and ensure peer feedback was constructive and returned feedback to TBL groups anonymously. Completing the peer evaluations with effortful and constructive feedback composed 5% of a student’s final grade.
Data Sources
Three quantitative measures of student outcomes in the course were analyzed. We analyzed final course grades, learning gains on the Biochemistry Concept Inventory (BCI) assessment (Villafañe et al., 2011), and students’ performance on unit exams. Final grades were selected because potential performance gaps could relate to subsequent course choice and success. The BCI was used as an external measure of student knowledge of biochemistry content. Unit exam scores were selected because of their potential to reveal performance gaps at discrete timepoints throughout the semester that might otherwise not be revealed when looking at aggregate exam performance. Performance on unit exams represents students’ experience at progressive timepoints during the semester. We examined unit exam performance to explore two possible predictions. One prediction was that no gaps or small gaps would exist in Exam 1 scores, but differences in students’ exam scores connected to demographic identities would increase over time as social interactions in TBL groups led to threats and exacerbated psychological barriers to performance. Another, coming from TBL literature that posits that groups improve as they learn to coordinate over time, would be that gaps would exist early in the semester, but would decrease over the semester due to groups creating positive norms and developing ways of working together (Watson et al., 1991; Letassy et al., 2008; Akl et al., 2012; Persky, 2012).
The course included two midterm exams and one comprehensive final exam. Exams consisted of upper-level Blooms questions and were mostly multiple-choice questions with some short-answer questions. In 2020, exams were administered online. That semester, time for the final exam was extended by one hour in accordance with university policies as an accommodation for COVID-19 conditions. The three exams together comprised 40% of a student’s grade in the course.
On the first and last days of class, the BCI was administered as a pretest and posttest. The students received points for completing the BCI with an effortful attempt, but their work was otherwise ungraded. Normalized change scores were calculated for each student using their BCI pretest and posttest scores and range from −1 to 1 (Marx and Cummings, 2007). The BCI normalized change score was used for analysis as it accounts for students who perform lower on the posttest than on the pretest, limiting negative outcomes from skewing further statistical analysis. Student exam scores and course grades had scores between 0 and 100.
Students’ self-identified demographic data were collected from requests to the registrar, and in some cases, a survey (IRB #19076). After these collections, the demographic information for some students was still not available. Those students’ data were excluded from the final models. The demographic makeups of the three semesters of the course, as well as the University, are listed in the categories used for modeling below (University: 2019 Fall).
The base, car, effsize, lme4, sjplot, and sjstats packages in R were used to test, model, and visualize the data.
Modeling Student Performance
We used the three performance measures to generate linear mixed models which included demographic, assessment, and grouping factors, as well as interactions between factors, as explanatory variables. We chose to use linear mixed models as they allowed us to examine student performance as explained by one factor or interaction between factors while controlling for the other factors of interest. Our analysis was informed by Theobald (2018). Model outcomes were generated using lme4, sjplot, and sjstats in R. Models incorporated the semester each student took the course (three total semesters), three factors describing student demographic information (gender, historically marginalized [HM] status, and generational status), and iRAT performance, as well as relevant interactions between gender and the other two demographic factors. Gender is not a binary construct; however, the demographic information was reported in a binary M-F format. Although transgender and nonbinary students were present in the course, and shared their identities with the instructors, they are not identified or separated in the data because of privacy concerns. HM or historically marginalized status is defined as a group of backgrounds including Black, Latino, Native Hawaiian, Pacific Islander, and Native American/Alaska Native racial/ethnic backgrounds. Students from these backgrounds disproportionately are affected by historical and current marginalization, resulting in underrepresentation in STEM. First-generation students are defined as those for whom none of their parents or guardians earned a bachelor’s degree. This definition of first-generation matches that used by the University where the Study took place.
We selected three demographic factors based on prior investigations of students from marginalized gender, racial/ethnic, and generational backgrounds in STEM courses with active learning practices (Hood et al., 2020; Theobald et al., 2020; Odom et al., 2021). Scholarly work explaining the interaction between students’ social identities and performance also contributed to our choice of factors, as all three have been correlated with threats to social identity in academic contexts (Easterbrook and Hadden, 2021). The interactions between the factors were selected due to their known joint effects on undergraduate experiences (Charleston et al., 2014; Laajala-Lozano and Jenkins, 2022). In initial models, we included interactions between gender and our other demographic factors of interest to account for intersectionality. Throughout the modeling process, these interaction factors were unable to explain student performance, were non-significant, and because of this were removed from the final models in the Exam 2, BCI normalized change, and course grades models.
Preparation has been a predictor of student performance in some active learning classrooms, so iRAT performance was selected as a factor as a proxy for students’ preparation before class (Gross et al., 2015). iRAT performance was also more strongly correlated with student exam scores than other potential factors (e.g., student BCI prescores, tRAT scores, team exercise scores; Supplemental Materials), so it was a better control for student work during the unit than those other factors. iRAT scores were removed from the final course grade calculation to prevent unintentional correlations. The factors we selected to represent students’ demographic backgrounds might logically be related as components of identity, so variance inflation factors were calculated between the selected independent variables to assess the degree to which they were correlated. This method suggested that the factors were not at risk of producing error (Supplemental Materials).
A backward selection method was used to build and test models (Theobald, 2018). First, the random factor (Semester) was tested by itself. Semester is a random factor in many models of student performance as students are nested within semesters, meaning they are not truly independent of each other (Theobald, 2018). In all models except for the comprehensive (third) exam model, the intraclass correlation coefficient (ICC) of Semester was 0 or near 0, meaning that Semester was not a significant factor in explaining student scores. In those models, modeling proceeded without the random factor, using only the fixed factors. In the Exam 3 model, the ICC of the Semester factor was far from 0, and modeling proceeded including Semester as a random factor. Then, models were tested with all fixed factors, and if factors were found not to be significant, new models were generated one by one with the factor furthest from significance removed, and the Akaike information criteria (AIC) of the new models compared to determine which model had the best fit to the data. If the AICs of the two models were significantly different (as denoted by a difference of 2 or more in the AICs of the two models), the model with the lower AIC (and thus the more efficient model) was used in further tests. This process continued with each non-significant factor until a model with only factors that were significant or significantly contributed to the AIC remained. Models were interpreted in order to address the research question concerning differences in performance in the classroom (Table 1).
Demographics | 2019 – University | 2019 – Course | 2020 – University | 2020 – Course | 2021 – University | 2021 – Course |
---|---|---|---|---|---|---|
Gender (Women) | 53.2% | 53.1% (17/32) | 54.4% | 50% (26/52) | 54.3% | 62.5% (30/48) |
HM status | 30.5% | 18.8% (6/32) | 31.5% | 30.8% (16/52) | 32.1% | 20.8% (10/48) |
First-generation | 32.9% | 25% (8/32) | 32.5% | 25% (13/52) | 31.9% | 16.7% (8/48) |
First-generation*Women | Not provided | 18.8% (6/32) | Not provided | 9.6% (5/52) | Not provided | 10.4% (5/48) |
Women*HM | Not provided | 9.4% (3/32) | Not provided | 11.5% (6/52) | Not provided | 10.4% (5/48) |
RESULTS
Exam Performance
The mixed models we generated to explain performance on Exams 1–3 show that the selected demographic characteristics did not explain any differences in performance (Tables 2–4). The only significant factor that explained exam performance in the initial models (Tables 2–4) was preparation (i.e., student average iRAT performance). The R2 values of the final exam models indicate that they had weak to moderate performance for explaining scores (Hair et al., 2011). Model outcomes were similar across exams, with iRAT performance explaining exam performance in each unit, and demographic factors unable to explain exam performance in any unit. There was no evidence of any cumulative effects or effects over time that affected student performance during the period of the course.
Factor | B | SE |
---|---|---|
Intercept | 36.78 | 6.06 |
iRAT average† | 0.57 | 0.07 |
Gender | −1.92 | 3.43 |
HM status | −0.84 | 3.09 |
Generation | 1.11 | 3.43 |
Gender*HM status | −1.56 | 4.52 |
Gender*Generation | −0.74 | 4.61 |
Fixed factors | B | SE |
---|---|---|
Intercept | 48.32 | 6.28 |
iRAT average† | 0.49 | 0.08 |
Gender | −3.23 | 2.21 |
HM status | −2.72 | 2.57 |
Generation | −3.41 | 2.59 |
Random factor | Variance | Std. Dev |
Semester | 12.76 | 3.57 |
Fixed factors | B | SE |
Intercept | 49.07 | 5.86 |
iRAT average† | 0.38 | 0.07 |
Gender | −2.43 | 2.22 |
HM status | −0.63 | 2.95 |
Generation | 1.61 | 3.01 |
Gender*HM status | 0.51 | 4.31 |
Gender*Generation | −6.45 | 4.15 |
Normalized Change and Course Grades
The models explaining normalized change on the BCI and course grades performed poorly compared with those explaining exam scores (Supplemental Materials). Students’ average iRAT scores across the course significantly explained a small amount of the BCI normalized change outcome (β = 0.006, Supplemental Materials). None of the selected factors significantly explained course grades. In further exploratory modeling, students’ BCI prescores were not able to explain student grades. The adjusted R2 values for these models were very low; indicating that the selected factors poorly explained the BCI normalized change scores and course grades.
DISCUSSION
TBL assigns students to permanent groups with maximum diversity to work intensely throughout the duration of a course (Watson et al., 1991; Letassy et al., 2008; Michaelsen and Sweet, 2008; Akl et al., 2012; Persky, 2012). We had hypothesized that the intense social interactions characteristic of TBL could produce threats to socially marginalized identities, the effects of which might be observed by differences in performance on internal, instructor-written exams, course grades, and/or external concept inventories. Our measures of marginalized student identity (gender, racial/ethnic group, generational status) could not statistically explain any difference in performance on any of these outcome variables. These results are encouraging because they suggest that, at a minimum, TBL did not introduce performance gaps in this upper-division course. This is an interesting result when juxtaposed to larger, meta-analyses of active learning which often show a reduction—but not elimination—of performance gaps.
Given the dataset collected, it is difficult to confidently explain this null result. Two potential explanations include survivorship bias and an inability to adequately account for invisible and intersectional identities. The context of this study was a single upper-division biochemistry course typically taken by students who are close to graduation. It is possible that our data suffer from a “survivorship bias” in the population of biochemistry majors. The concept of “survivorship bias” reflects the inability for datasets to include information from subjects who have already left the sample population by the time data can be collected (Brown et al., 1992). Students with socially marginalized identities may have already experienced disproportionate course failures, withdrawals, major changes, and exit from the University before advancing to this upper-division course. Indeed, the biochemistry course had fewer students from HM and first-generation backgrounds than the university as a whole (Demographics Table). Due to their early success in lower-division courses, the remaining students who reached their senior year may have developed greater levels of self-efficacy, providing a protective effect against identity threats. Because our study focused on performance and not other constructs thought to be influenced by the use of TBL like self-efficacy, engagement, or motivation, we were not able to investigate the interaction between the backgrounds we focused on, the TBL context, and these constructs.
Intersectionality is a crucial concept in understanding equity in STEM teaching and learning because it acknowledges how individuals can hold multiple identities at once, and for individuals with multiple stigmatized identities, different forms of discrimination can combine to create major obstacles to progression in STEM, especially for women of color (Ong et al., 2011). We were limited in our examination of intersectionality due to the course makeup and sample size. Just 16 students were first-generation women students, and just 14 students were women from underrepresented racial/ethnic backgrounds. While our sample was enough to conduct an analysis, it limited the statistical power of our attempts to model interactions among these characteristics or between other combinations of characteristics. Further, our predictor variables were limited to those routinely collected by the institution. We did not have measures for other identities that have been marginalized in educational contexts (e.g., disability, LGBTQ identity) (Cooper and Brownell, 2016; Cooper et al., 2018; Easterbrook and Hadden, 2021; Marques, 2022; Pfeifer et al., 2023). Finally, we were unable to account for invisible identities for which there are no convenient measures (e.g., neurodiversity, religiosity). It is possible that performance could have been explained by a more comprehensive set of variables that better account for a broader diversity of identities and their intersections. Exploring these other identities may reveal differences in performance that could not be detected in our study, and thus lead to additional opportunities to understand how positive group dynamics can be promoted in TBL courses that have diverse groups of students along those identities as well.
CONCLUSIONS AND FUTURE DIRECTIONS
This study is the first that has documented comparable performance outcomes among students from diverse identity backgrounds in a TBL context in the undergraduate biosciences. The extent of differences in performance between students with marginalized identities and their advantaged peers during TBL has been underexamined in the literature, and our work investigated just one of many contexts where TBL takes place. To more fully understand the degree that TBL impacts student performance requires studies that look at disaggregated performance effects of TBL in different undergraduate contexts. Additionally, future work should investigate other outcomes (e.g., motivation, engagement, self-efficacy) that also effect student success.
TBL is just one form of active learning. An extensive body of research demonstrates that active learning reduces, but does not completely close, gaps in performance between students of marginalized and advantaged backgrounds (Theobald et al., 2020). In our analysis of this one TBL context, we were not able to detect any gaps in performance. The multiple caveats of our research design, as well as the many facets of students’ identities we were not able to explore, preclude us from claiming such gaps do not exist in TBL contexts, but they encourage us to call for more examination of TBL as a practice. Further work with stronger controls, deeper disaggregation, and more robust replication is necessary to truly determine the extent to which TBL may reduce or eliminate performance gaps among students from different backgrounds. More evidence indicating TBL reduces or even eliminates gaps in performance would be a major advancement for STEM educators desiring equitable practices for their increasingly diverse courses.
ACKNOWLEDGMENTS
The authors would like to acknowledge Dr. Jessie Arneson and the WSU Center for Interdisciplinary Statistical Education and Research for their assistance with the conception and methods of analysis for this research. Funding for this project was provided by a National Science Foundation Graduate Research Fellowship (Award ID: 1842493)